Abstract
Nowadays, machine learning projects have become more and more relevant to various real-world use cases. The success of complex Neural Network models depends upon many factors, as the requirement for structured and machine learning-centric project development management arises. Due to the multitude of tools available for different operational phases, responsibilities and requirements become more and more unclear. In this work, Machine Learning Operations (MLOps) technologies and tools for every part of the overall project pipeline, as well as involved roles, are examined and clearly defined. With the focus on the inter-connectivity of specific tools and comparison by well-selected requirements of MLOps, model performance, input data, and system quality metrics are briefly discussed. By identifying aspects of machine learning, which can be reused from project to project, open-source tools which help in specific parts of the pipeline, and possible combinations, an overview of support in MLOps is given. Deep learning has revolutionized the field of Image processing, and building an automated machine learning workflow for object detection is of great interest for many organizations. For this, a simple MLOps workflow for object detection with images is portrayed.
1. Introduction
Across multiple industries, new applications and products are becoming increasingly Machine Learning (ML)-centric. In general, ML is still a tiny part of a larger ecosystem of technologies involved in an ML-based software system. ML systems, i.e., a software system incorporating ML as one of its parts, are known to add several new aspects previously not known in the software development landscape. Furthermore, manual ML workflows are a significant source of high technical debt [1]. Unlike standard software, ML systems have complex entanglement with the data on top of standard code. This complex relationship makes such systems much harder to maintain in the long run. Furthermore, different experts (application developers, data scientists, domain engineers, etc.) have to work together. They have different temporal development cycles and tooling, and data management is becoming the new focus in ML-based systems. As a reaction to these challenges, the area of Machine Learning Operations (MLOps) emerged. MLOps can help reduce this technical debt as it promotes automation of all the steps involved in the construction of an ML system from development to deployment. Formally, MLOps is a discipline which is formed of a combination of ML, Development Operations (DevOps), and Data Engineering to deploy ML systems reliably and efficiently [2,3].
Advances in deep learning have promoted the broad adoption of AI-based image analysis systems. Some systems specialize in utilizing images of an object to quantify the quality while capturing defects such as scratches and broken edges. Building an MLOps workflow for object detection is a laborious task. The challenges one encounters when adopting MLOps are directly linked with the complexity due to the high dimensionality of the data involved in the process.
Data are a core part of any ML system, and generally, they can be divided into three categories, i.e., structured, semi-structured, and unstructured data. Vision data, i.e., images and videos, are considered as unstructured data [4]. Analyzing images and videos by deep neural networks (DNNs) like FastMask has gained much popularity in the recent past [5]. Nevertheless, processing images with ML is a resource-intensive and complex process. As the training of DNNs requires a considerable amount of data, automated data quality assessment is a critical step in an MLOps workflow. It is known that if the quality of data is compromised to some specific level, this makes a system more prone to failure [6]. For images, it is challenging to define metrics for automated quality assessment because metrics such as completeness, consistency, etc., cannot be universally defined for image data sets. On the other hand, structured data are easier to process as one can clearly define data types, quality ratings (set by domain experts), and quality assessment tests.
Moreover, several tools are available for building an automated MLOps workflow. These tools can be used to achieve the best outcomes from developing of a model to deployment until maintenance. In most cases creating an MLOps workflow requires multiple tools which collaborate to fulfill individual parts.
There is also a high overlap of functionalities provided by many of these tools. The selection of tools for an optimal MLOps pipeline is a tedious process. The recipe for the selection of these tools is the requirements that each step of the workflow introduces. This recipe is also dependent on the maturity level of the machine learning workflow adopted in an organization and the capabilities of integration of each tool or ingredient. Therefore, we address these challenges in the work on hand. The main contributions of this paper are as follows:
-
A holistic analysis and depiction of the need for principles of MLOps.
-
An intensive consideration of related roles in MLOps workflows and their responsibilities.
-
A comprehensive comparison of different supportive open-source MLOps tools, to allow organizations to make an informed decision.
-
An example workflow of object detection with deep learning that shows how a simple GitFlow-based software development process can be extended for MLOps with selected MLOps tools.
Demystifying MLOps and selection procedure of tools is of utter importance as the complexity of ML systems grows with time. Clarification of stages, roles, and tools is required so that everyone involved in the workflow can understand their part in the development of whole system.
This paper is structured as follows. In Section 2, a quick overview of work mentioning and using MLOps techniques is given and is followed by the depiction of workflows in MLOps in Section 3. With a description of the various roles in Section 4 and a comparison of MLOps supporting tools and applicable monitoring metrics in Section 5, a use case for automating the workflow for object detection with deep neural nets is discussed in Section 6. With potential future research directions, this work is concluded in Section 7.
2. Related Work and State-of-the-Art
As MLOps is a relatively new phenomenon, there is not enough related work, implementation guidance, specific instructions for starting such project structures, or experience reports. Therefore, we outline work on different aspects of MLOps in the following, paying particular attention to holistic MLOps workflows, data quality metrics, and ML automation. By addressing these core aspects during the work on hand, we aim to clarify the potentials and practical applications of MLOps.
Trends and challenges of MLOps were summarized by Tamburri in [7]. In this paper, MLOps is defined as the distribution of a set of software components realizing five ML pipeline functions: data ingestion, data transformation, continuous ML model (re-)training, (re-)deployment, and output presentation. By further discussing trends in artificial intelligence software operations such as explanation or accountability, an overview of state-of-the-art in MLOps is given. While the author defined and discussed trends and challenges in sustainable MLOps, the work at hand also covers a comparative overview of responsibilities and possible tool combinations concerning image processing. A framework for scalable fleet-analytic (e.g., ML in distributed systems) was proposed by Raj et al. in [3], facilitating ML-Ops techniques with focus on edge devices in Internet of Things (IoT). The proposed scalable architecture was utilized and evaluated concerning efficiency and robustness in experiments covering air quality prediction in various campus rooms by applying the models on the dedicated edge devices. As the formulated distributed scenario underlines the demand for a well-defined MLOps workflow, the authors briefly introduced the applied modeling approach. Next to the focus on IoT devices, the work on hand differs due to an extensive discussion of applicable metrics, utilization of tools while experimenting, and a definition of involved actors and their responsibilities. Fursin et al. proposed an open platform CodeReef for mobile MLOps in [8]. The non-intrusive and complementary concept allows the interconnection of many established ML tools for creating specific, reproducible, and portable ML workflows. The authors aimed to share various parts of an MLOps project and benchmark the created workflow within one platform. In contrast, this work considers multiple applicable solutions to the various steps in such workflows. Two real-world multi-organization MLOps setups were presented by Granlud et al. in [9]. With a focus on use cases that require splitting the MLOps process between the involved organizations, the integration between two organizations and the scaling of ML to multi-organizational context were addressed. The physical distribution of ML model training and the deployment of resulting models are not explicitly responded to by the work on hand. However, one can assemble its environment boundaries, dependent on the selected toolchain. Although both scenarios stated by the authors were highly domain-specific, the elementary formulation and differentiation of ML and deployment pipelines overlap with this work. Zhao et al. reviewed the literature of MLOps. Briefly, they identified various challenges of MLOps in ML projects, differences to DevOps, and how to apply MLOps in production in [10]. Muralidhar et al. summarized commonly practiced antipatterns, solutions, and future directions of MLOps in [11] for financial analytics. In applying the stated recommendations, error sources in ML projects can be reduced. Next to conforming with the stated collateral best practices in MLOps workflows, the work on hand also tries to extend and generalize the author’s definition of involved actors, specific to the financial use case.
An approach for easy-to-use monitoring of ML pipeline tasks, while also considering hardware resources, was presented by Silva et al. in [12]. Concerning the required time for completing tasks (e.g., operations in batch or stream processing data sets) and focus on resource utilization, thoughts on benchmarking ML-based solutions in production were given. The authors overall compared nine datasets consistent with binary and multiclass regression problems, where three datasets were based on images. Their approach was evaluated by benchmarking all datasets in batch mode and applying five datasets for online tasks with every 50 and 350 threads. Monitoring aspects of resources in the different stages of an MLOps workflow is included in the work on hand, and there is no focus on benchmarking the chosen toolchain environment. Concerning data warehousing applications, Sureddy and Yallamula proposed a monitoring framework in [13]. In defining various requirements for monitoring such complex and distributed systems, the framework helps in building effective monitoring tools. As MLOps systems require a monitoring solution, too, aspects of monitoring the server as well as the application are treated in the work on hand. Various aspects and best practices of monitoring the performance and planning infrastructure for specific projects were outlined by Shivakumar in [14]. In considering the server- as well as the application side of such undertakings, a CICD sample setup and sample strategy of using commercial tools for infrastructure monitoring in a disaster recovery scenario were given. As the monitoring and CICD aspects are an inevitable part of MLOps, the work on hand is not concerned with disaster recovery. A definition of measuring data quality dimensions as well as challenges while applying monitoring tools was outlined by Coleman in [15]. By translating user-defined constraints into metrics, a framework for unit tests for data was proposed by Schelter et al. in [16]. Using a declarative Application Programming Interface (API) which consumes user-defined validation codes, the incremental- and batch-based assessment of data quality was evaluated on growing data sets. Regarding the data completeness, consistency, and statistics, the authors proposed a set of constraints and the respective computable quality metrics. Although the principles of a declarative quality check for datasets are applicable during an MLOps workflow, the enumeration of this approach is a surrogate for the definition of quality check systems and services.
Taking the big data value chain into consideration, there are similar requirements to the domain of ML quality. Various aspects of demands on quality in big data were surveyed by Taleb et al. in [17] and answered by a proposed quality management framework. While considering the stated demands on data quality during the various sections of the work on hand, no specific framework or quality management model for big data value chains, as introduced by the authors, is proposed. Barrak et al. empirically analyzed 391 open-source projects which used Data Version Control (DVC) techniques with respect to coupling of software and DVC artifacts and their complexity evolution in [18]. Their empirical study concludes that using DVC versioning tools becomes a growing practice, even though there is a maintenance overhead. As DVC is a part of the work on hand, it neither exclusively focuses on versioning details nor takes repository and DVC-specific statistics into consideration. Practical aspects of performing ML model evaluation were given by Ramasubramanian et al. in [19] concerning a real life dataset. In describing selected metrics, the authors introduced a typical model evaluation process. While the utilization of well-known datasets is out of scope for the work on hand, the principles of ML model evaluation are picked up during the various subsequent sections. A variety of concept drift detection techniques and approaches were evaluated by Mhemood et al. in [20] with respect to time series data. While the authors gave a detailed overview of adaption algorithms and challenges during the implementation, aspects of monitoring the appearance of concept drift are picked up in work on hand. The various methods, systems, and challenges of Automated Machine Learning (AutoML) were outlined in [21]. Concerning hyperparameter optimization, meta-learning, and neural architecture search, the common foundation of AutoML frameworks is described. Subsequently, established and popular frameworks for automating ML tasks were discussed. As the automation of the various parts in an ML pipeline becomes more mature, and the framework landscape for specific problems grows, the inclusion of ML-related automation in MLOps tasks becomes more attractive. The area of AutoML was surveyed by Zöller et al. in [22]. A comprehensive overview of techniques for pipeline structure creation, Combined Algorithm Selection and Hyperparameter optimization (CASH) strategies, and feature generation is discussed by the authors. As the AutoML paradigm has the potential of performance loss while training model candidates, different patterns of improving the utilization of such systems are introduced. By discussing the shortcomings and challenges of AutoML, a broad overview of this promising discipline was given by the authors. Although we refer to specific aspects of ML automation in work on hand, models’ deployment and integration into the target application are treated more intensely. Concerning research data sets, Peng et al. provided international guidelines on sharing and reusing quality information in [23]. Based on the Findable, Accessible, Interoperable, and Reusable (FAIR) principles, different data lifecycle stages and quality dimensions help in systematically processing and organizing data sets, their quality information, respectively. While determining and monitoring the quality of datasets and processed data structures are vital to different operations in MLOps, the work on hand does not address the sharing of preprocessed records. A systematic approach for utilizing MLOps principles and techniques was proposed by Raj in [24]. With a focus on monitoring ML model quality, end-to-end traceability, and continuous integration and delivery, a holistic overview of tasks in MLOps projects is given, and real-world projects are introduced. As the authors focused on practical tips for managing and implementing MLOps projects using the technologies Azure in combination with MLflow, the work on hand considers a broader selection of supportive frameworks and tools. Considering automation in MLOps, various roles and actors’ main tasks are supported by interconnected tooling for the dedicated phases. Wang et al. surveyed the degree of automation required by various actors (e.g., 239 employees of an international organization) in defining a human-centric AutoML framework in [25]. With visualizing the survey answers, an overview of the different actor’s thoughts on automating the various phases of end-to-end ML life cycles was given and underlined the author’s assumption of only partly automating processes in such complex and error-prone projects.

Additionally, the landscape of MLOps tools has evolved massively in the last few years. There has been an emergence of high-quality software solutions in terms of both open-source and commercial options. The commercial platforms and tools available in the MLOps landscape make ML systems development more manageable. One such example is the AWS MLOps framework [26]. The framework is one of the easiest ways to get started with MLOps. The framework is built on two primary components, i.e., first, the orchestrator, and second, the AWS CodePipeline instance. It is an extendable framework that can initialize a preconfigured pipeline through a simple API call. Users are notified by email about the status of the pipeline. There are certain disadvantages of using commercial platforms for MLOps. The development process requires multiple iterations, and you might end up spending much money on a solution that is of no use. Many training runs do not produce any substantial outcome. To have a flexible and evolving workflow, it is essential to have a 100% transparent workflow, and with commercial solutions, this can not be completely ensured. In general, open-source tools are more modular and often offer higher quality than their counterparts. This is the reason why only open-source tools are benchmarked in this paper.
3. Workflow of DevOps and MLOps
With the development of hardware for the efficient development of ML systems [24], like GPUs and TPUs, software development has evolved with time. DevOps has been revolutionary for achieving this task for traditional software systems, and similarly, MLOps aims to do this for ML-centered systems. In this section, the essential terminology for DevOps and MLOps is explained.
3.1. DevOps Software Development
Software development teams have moved away from the traditional waterfall method for software development to DevOps in the recent past [24]. The waterfall method is depicted in Figure 1a. The method comprises five individual stages: Requirement Analysis, Design, Development, Testing, and Maintenance. Every individual stage of the cycle is pre-organized and executed in a non-iterative way. The process is not agile, and thus all the requirements are collected before the cycle begins, and modification in requirements is impossible. Once the requirements are implemented (Development stage), then only the testing can begin. As soon as the testing is complete, the software is deployed in production for obtaining user feedback. If, in the end, the customer is not satisfied, then the whole pipeline is repeated. Such a life cycle is not suited for dynamic projects as needed in the ML development process. To counter these disadvantages, the whole process has evolved into an agile method. Unlike the waterfall method, the agile development process is bidirectional with more feedback cycles such that the immediate changes in requirements can be incorporated faster in the product. The agile method is aimed at producing rapid changes in code as per the need. Therefore, close collaboration between Ops and software development teams is required.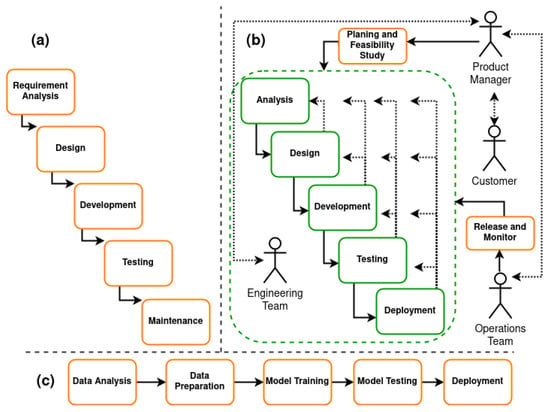
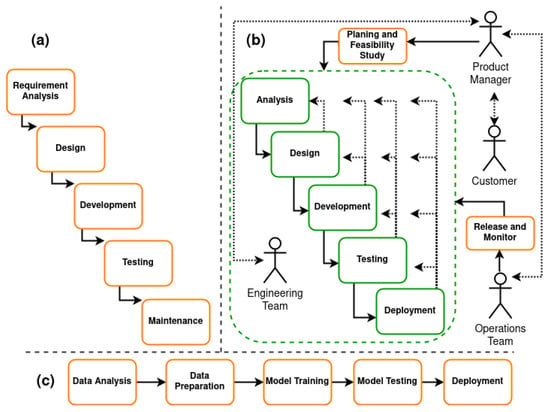
Figure 1. (a,b) Difference between Waterfall and DevOps software development life cycle. (c) A manual ML Pipeline.
3.2. Dev vs. Ops
After the agile methodology, DevOps emerged as the new go-to methodology for continuous software engineering. The DevOps method extended the agile development method by streamlining the flow of software change through the build, test, deploy, and delivery stages [24]. Looking at Dev and Ops individually, there was hardly any overlap between them. In the past, software development consisted of two separate functioning units, i.e., Dev (Software engineers and developers) and Ops (Operations engineers and IT specialists). The Dev was responsible for translating the business idea into code, whereas the Ops were responsible for providing a stable, fast, and responsive system to the customer at all times [27].
3.3. DevOps
Traditionally, the developers would wait until the release date to pass the newly developed code (patch) to the operations team. The operations team would then foresee that the developed code is deployed with additional infrastructure abstraction, management, and monitoring tasks. In contrast, DevOps aimed at bridging the gap between the two branches: Dev and Ops. It responds to the agile need by combining cultural philosophies, practices, and tools that focus on increasing the delivery of new features in production. It emphasizes communication, collaboration, and integration between Software Developers and Operations team [27]. An example of a DevOps workflow is depicted in Figure 1b. As seen from the Figure, the customer and project manager can redirect the development team on short notice if there are any changes in the specification. The different phases of DevOps can be implemented in a shorter duration such that new features can be deployed rapidly. The prominent actors involved in the DevOps process are also depicted in Figure 1b.
DevOps has two core practices: Continuous Integration (CI) and Continuous Delivery (CD). Continuous Integration is a software practice that focuses on automating the process of code integration from multiple developers. In this practice, the contributors are encouraged to merge their code into the main repository more frequently. This enables shorter development cycles and improves quality, as flaws are identified very early in the process. The core of this process is a version control system and automated software building and testing process. Continuous Delivery is a practice in which the software is built in a manner that is always in a production-ready state. This ensures that changes could be released on demand quickly and safely. The goal of CD is to get the new features developed to the end user as soon as possible [27,28].
There is also another practice known as Continuous Deployment, which is often confused with CD. Continuous deployment is a practice in which every change is deployed in production automatically. However, some organizations have external approval processes for checking what should be released to the user. In such cases, Continuous delivery is considered a must, but Continuous deployment is an option that can be left out.
3.4. CI and CD Pipeline
CI and CD have been adopted as the best practices for software development in recent years. Automating these practices also requires a robust pipeline known as the DevOps pipeline or CI/CD pipeline. The pipeline consists of a series of automated processes that enable software teams to build and deploy a new version of software smoothly. It ensures that the new developments are automatically merged into the software, which is followed up by automated testing and deployment. As a fact, the DevOps with CI and CD has helped in improving and accelerating deployments of new features into production [29].
3.5. ML and ML Pipeline
An ML system is also a software system, but the development of an individual ML model is essentially experimental. A typical ML workflow starts by gathering more insights about the data and the problem at hand. The data need to be analyzed (Exploratory data analysis (EDA)), cleaned, and preprocessed (feature engineering and selection) such that essential features are extracted from the raw data (with the support of Data Stewards). This is followed by dataset formation for training, testing, and validation.
Training, validating, and testing ML algorithms is not a straightforward method. The iterative process involves fitting the hyperparameters of an ML algorithm on the training data set while using the validation set to check the performance on the data that the model has not seen before at every iteration. Finally, the test dataset is used to assess the final, unbiased performance of the model. The validation dataset is unique concerning the test dataset because it is not kept hidden from the preparation of the model, however it is instead used to give an adequate performance of the ability of the last tuned model.
At the very beginning only, these three sets of data are separated and should not be mixed at any point in time. Once the dataset is ready for training, the next step is algorithm selection and, finally, training. This step is iterative, where multiple algorithms are tried to obtain a trained model. For each algorithm, one has to optimize its hyperparameters on the training set and validate the model on the validation set, which is a time-consuming task (and implemented by a data scientist). Multiple iterations are required for acquiring the best model, and keeping track of all iterations becomes a hectic process (often, this is manually done in excel sheets). To regenerate the best results, one must use the precise configuration (hyperparameters, model architecture, dataset, etc.). Next, this trained model is tested using specific predefined criteria on the test set, and if the performance is acceptable, the model is ready for deployment. Once the model is ready, it is handed over to the operations team, which handles the deployment and monitoring process (usually implemented by an Operation engineer) such that model inference can be done.
In the above process, all steps are incremental (similar to the waterfall software development style). These steps together form an ML pipeline as shown in Figure 1c. An ML pipeline is a script-based, manual process formed of a combination of tasks such as data analysis, data preparation, model training, model testing, and deployment. There is no automatic feedback loop and transitioning from one process to another is also manually done [29].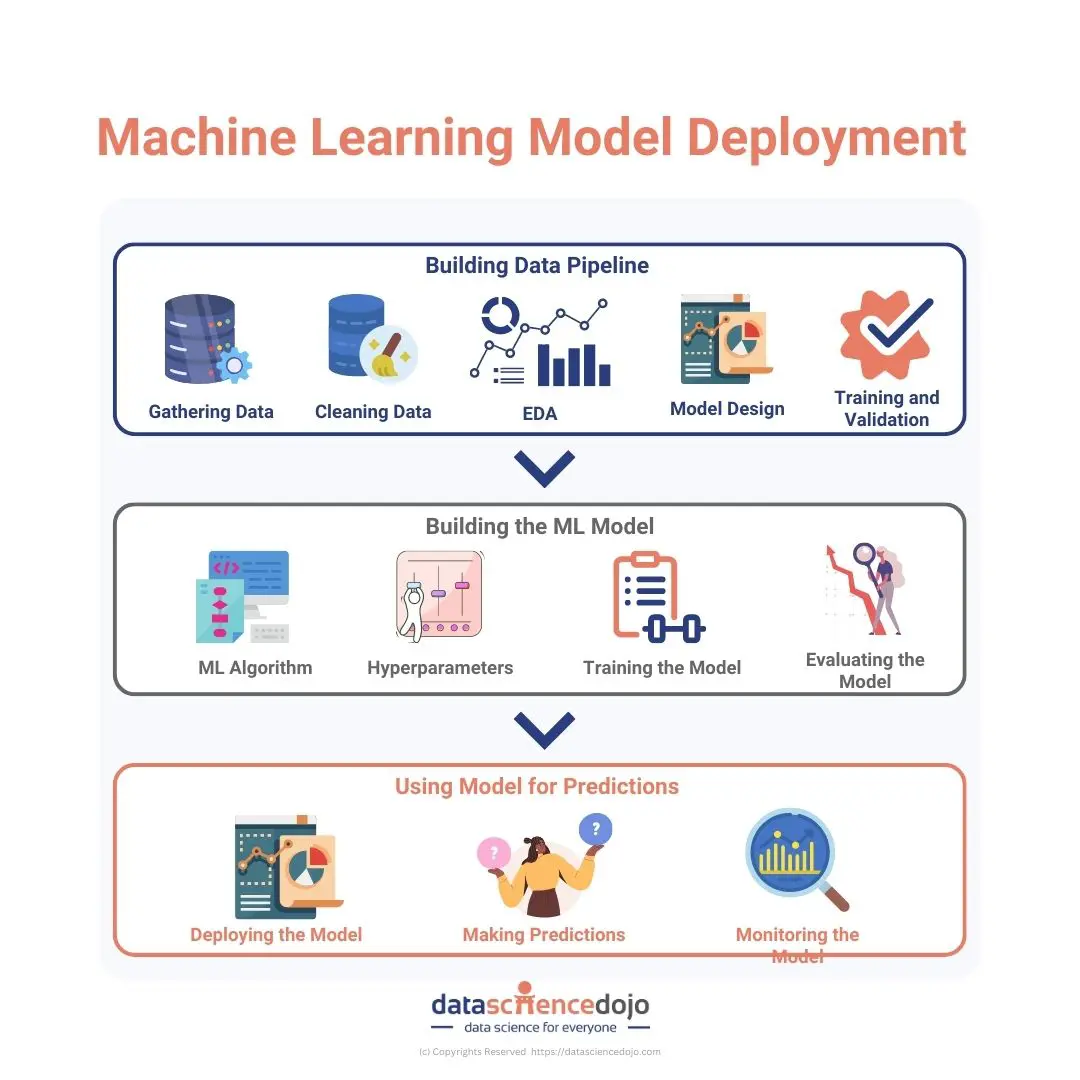
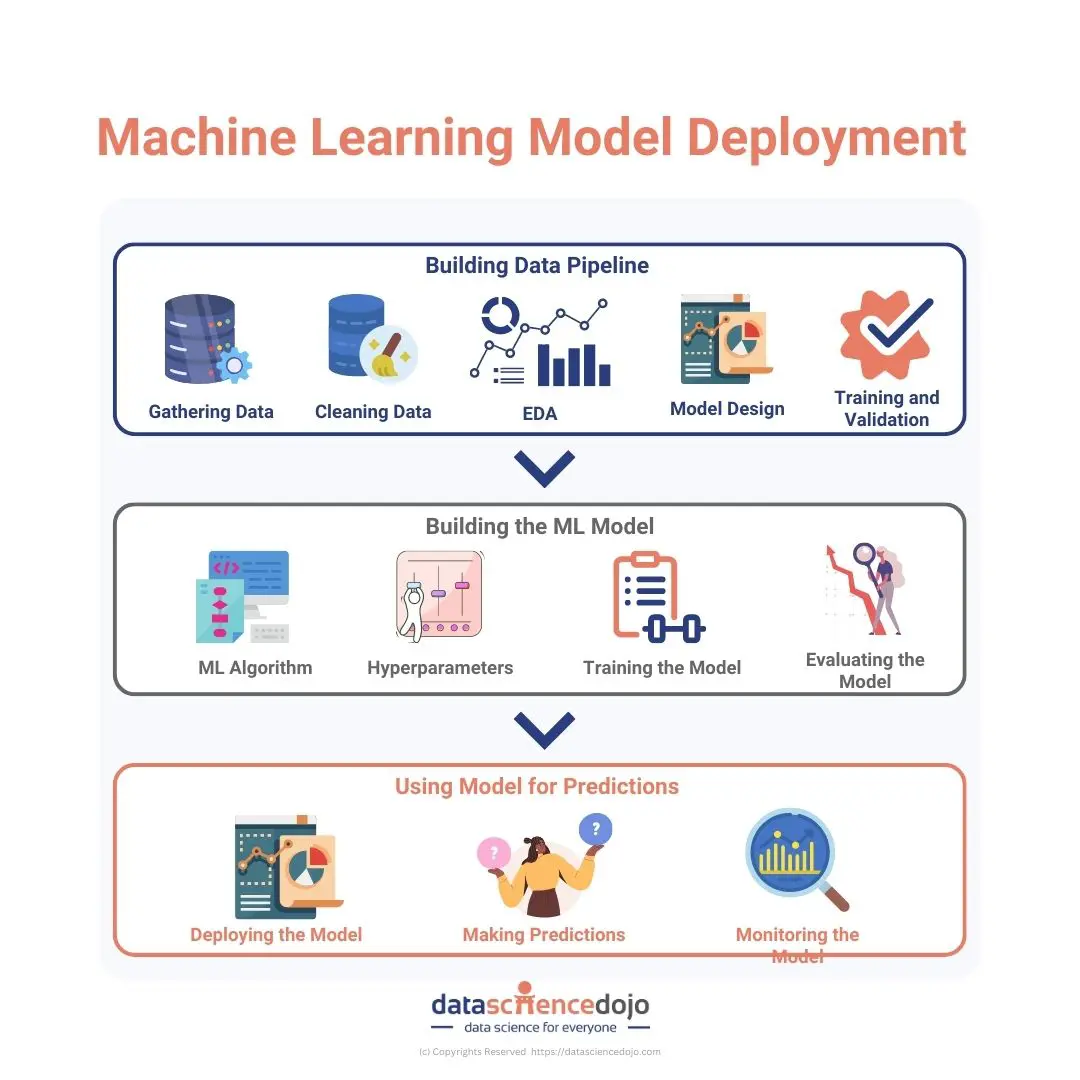
3.6. Operations in ML
Building an ML model is a role designated for a data scientist with the support of a Domain expert, and it does not intersect with how the business value is produced with that model. In a traditional ML development life cycle, the operations team is responsible for deploying, monitoring, and managing the model in production. Once the data scientist implements the suitable model, it is handed over to the operations team.
There are different techniques in which a trained model is deployed. The two most common techniques are “Model as a Service” and “Embedded model” [30]. In “Model as a Service”, the model is exposed as Representational state transfer (REST) API endpoints, i.e., deploying the model on a web server so that it can interact through REST API and any application can obtain predictions by transferring the input data through an API call. The web server could run locally or in the cloud. On the other hand, in the “Embedded model” case, the model is packaged into an application, which is then published. This use case is practical when the model is deployed on an edge device. Note that how an ML model should be deployed is wholly based on the final user’s interaction with the output generated by the model.
3.7. Challenges of Traditional ML Workflow
In comparison to conventional software development, ML has unique challenges that need to be handled differently. The major challenges encountered in the manual ML pipeline are as follows.
-
ML is a metrics-driven process, and in order to select the best model, multiple rounds of experiments are performed. For each experiment, one needs to track the metrics manually which increases the overall complexity.
-
Manual logging is an inefficient and error-prone process.
-
Data are not versioned. This further adds to the complexity as to reproduce results not only code, but data is also required. Similar to code, data also evolve.
-
No model versioning or model registry is available. It is harder to reproduce a model from the past.
-
Code reviews are not performed in ML. Testing is missing, such as unit or integration tests are not performed, which are commonly seen in traditional software development. The code development is limited to individual development environments, such as jupyter notebooks, on a data scientist’s local workstation.
-
The end product of the manual process is a single model rather than the whole pipeline.
-
Collaboration between team members is a headache as it is difficult to share models and other artifacts.
-
There is neither CI nor CD in the workflow as operations and development are considered as two distinct branches of the whole process.
-
There is a lack of reproducibility as the experiments and the models produced (artifacts) are not tracked. There is no concept of maintenance of experiments.
-
The deployed Model can not be retrained automatically, which might be required due to a number of reasons such as model staleness or concept drift. As the process is manual, deploying a new model takes a lot of time and there are less frequent releases.
-
Changing one particular parameter (for example, a specific hyperparameter that impacts the dimensions in a deep neural network) changes the total pipeline and influences the pipeline stages afterward and may lack versioning or may contradict.
3.8. MLOps Workflow
Building an ML pipeline is a strenuous task. The whole pipeline is often built sequentially with the help of tools that hardly integrate. The MLOps aims to automate the ML pipeline. It can be thought of as the intersection between machine learning, data engineering, and DevOps practices [24]. Essentially, it is a method for automating, managing, and speeding up an ML model’s lengthy operationalizing (build, test, and release) by integrating the DevOps practices into ML.
DevOps practices mentioned in the previous section, such as CI and CD, have helped keep the software developers and operations team in one loop. Through their continuous integration, new reliable features are added to the deployed product more rapidly. MLOps perceives the motivation from DevOps as it also aims to automate ML model development and deployment process.
In addition to integrating CI and CD into machine learning, MLOPs also aim to integrate a further practice known as CT (Continuous Training), which accounts for the variation between traditional software development and ML. As in ML, the performance of the model deployed might degrade with time due to specific phenomena such as concept drift, and data drift [31]. There is a need to retrain the already deployed model on newer incoming data or deploy a completely new model in production. This is where CT comes to the rescue as it aims to retrain and deploy the model automatically whenever the need arises. CT is the requirement for obtaining high-quality predictions all the time [32,33].
MLOps is a relatively new discipline. Therefore, there is not a fixed or standardized process through which MLOps is achieved. As there are different levels of automation with any workflow, so is also the case with MLOps, as discussed in [33]. Level-0 is the one where one starts to think about automating the ML workflow. The first aim is to achieve continuous training of the model to have a continuous delivery in production. On top of this, there should be a facility for automated data validation, code validation, experimentation tracking, data, and model versioning. This marks the completion of MLOps level-1, where an automated workflow is executed through some triggering mechanism. Furthermore, there could be more automated steps in the above workflow, e.g., deploying a production-ready pipeline itself rather than just the model that embarks the transition into the final stage or Level-2 of MLOps [33].
With the help of the first level of automation, one can get rid of the manual script-driven ML workflow that is highly prone to error. It also lays the path for a more modular and reusable structure that can be easily altered according to changes in requirements. However, at this point, new pipelines are not developed rapidly, and the development team is limited to just a few pipelines. The testing of these pipelines and their parts is also done manually. Once the tests are done, then the pipeline is handed over to the operations team. The pipeline is executed sequentially, and each part of the pipeline strongly depends on its predecessors. If the data processing part (relatively early part of the pipeline) fails, there is no need to run the rest of the pipeline. Thus, there is a need for pipeline versioning where the whole pipeline is deployed and versioned together. This is achieved in the final level of MLOps automation. Through this, many robust pipelines are managed. It promotes more collaboration between the data scientist and operations team. An essential part of the final stage is CI/CD pipeline automation to achieve a robust automated ML development workflow.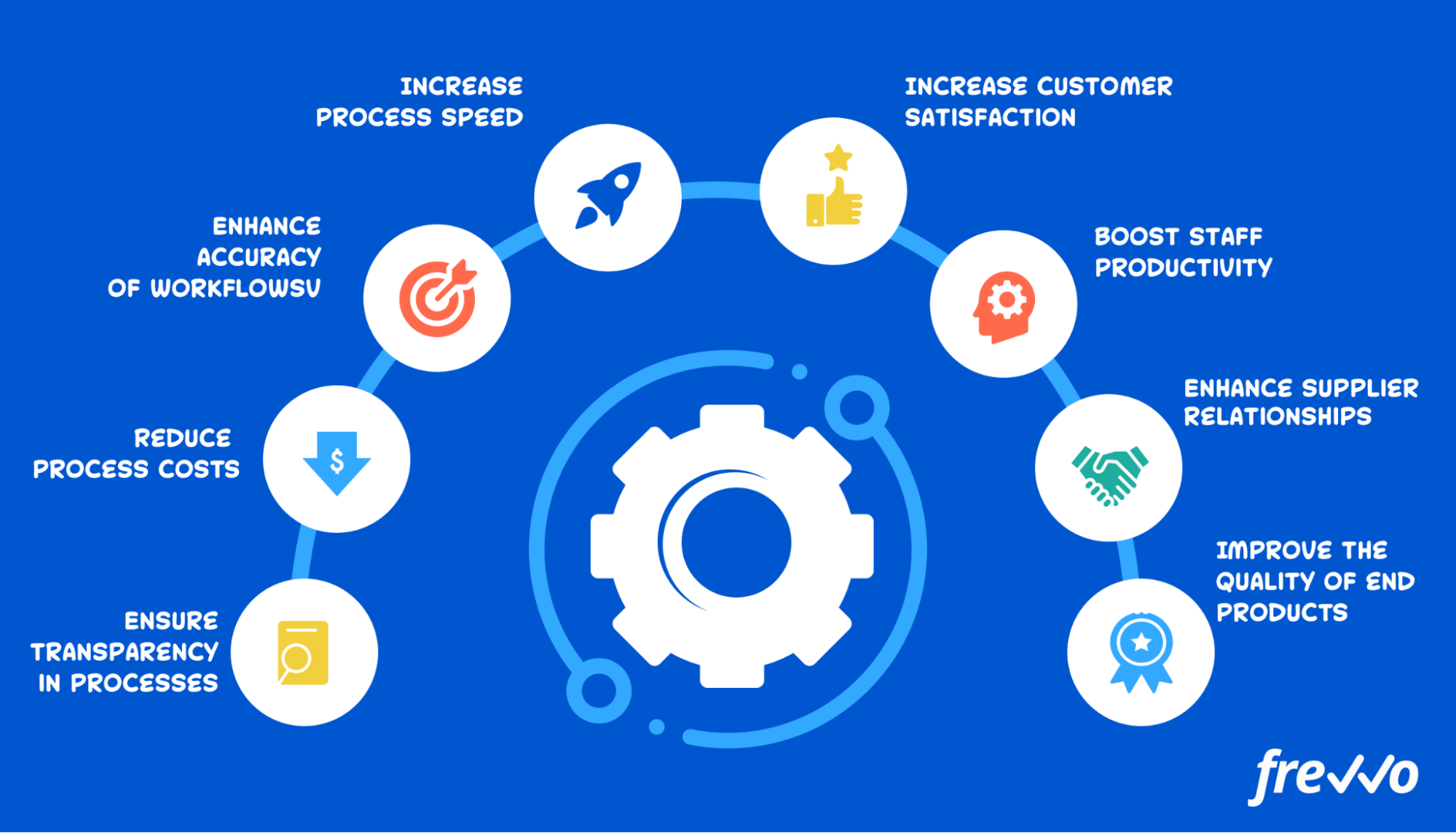
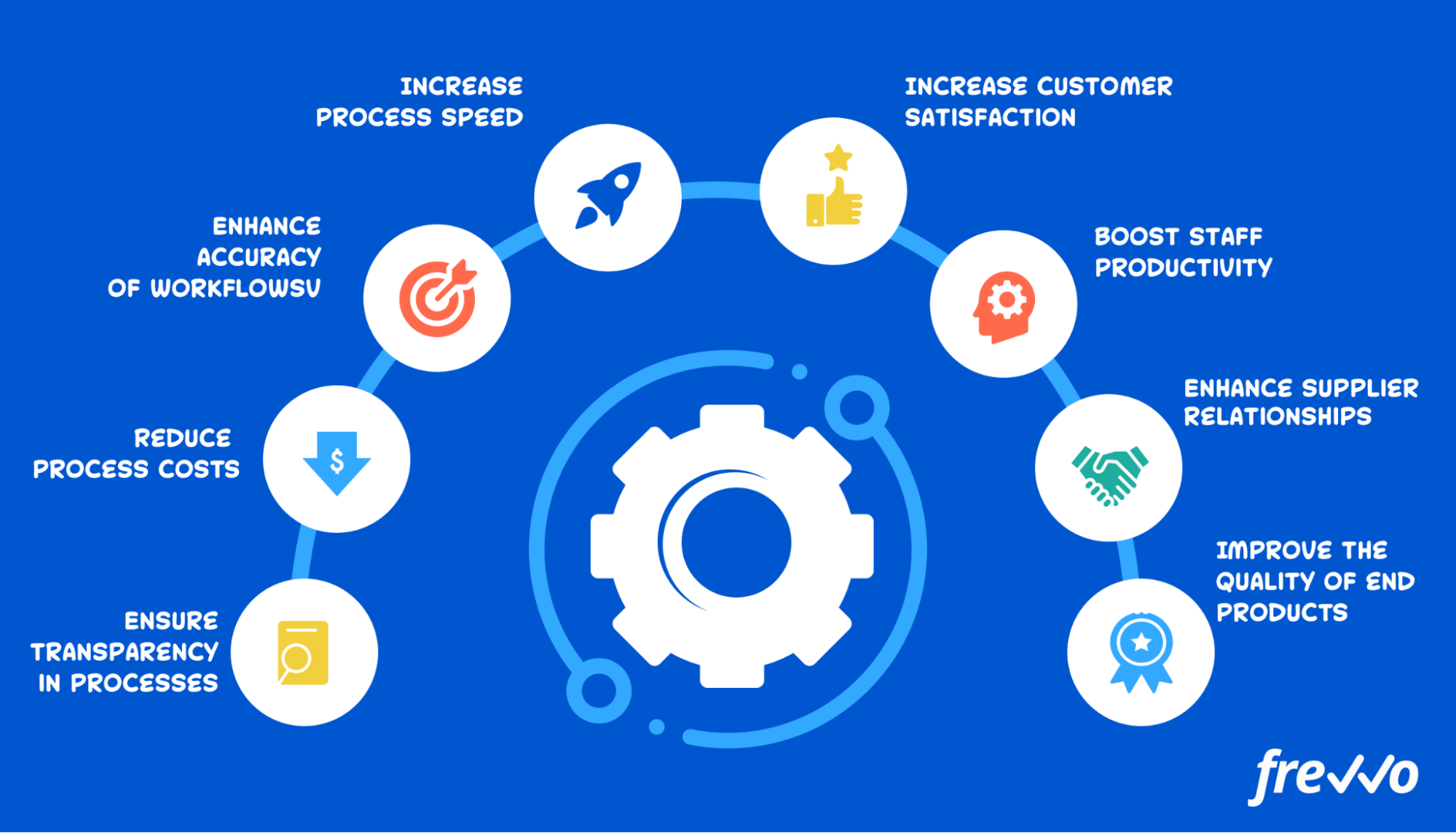
4. Workflow Stages in MLOps
Throughout the whole MLOps project, risk managers and auditors minimize model-caused risks and ensure compliance with the predefined project requirements [30]. As depicted in Figure 2, each role involved in an MLOps scenario may influence various steps among the workflow of implementing the solution. The task of deciding which stage should be fulfilled by which actor is not so trivial as designing an ideal MLOps workflow; multiple iterations are required. For organizations that are freshly adopting MLOps, there is a need to clarify the involvement of actors in the different stages. Reasoning from this, first, a brief listing of different roles involved in MLOps is depicted. In assembling related working packages into the different MLOps phases, e.g., Data Management, ML Preparation, and ML Training and Deployment, the responsibilities of each actor are described in more detail in the following. Additionally, requirements on supportive tools of the respective phases (as further described in Section 5.1) are referenced. Finally, for each phase, we define a section as Supportive Tool Requirements, which outline the recipes or features needed to be fulfilled in that phase. Subsequently, we outline each component in detail for that particular stage. The involved actors of MLOps workflow are as follows:
-
Data Scientists are involved in various phases of MLOps, such as designing the feasibility of elementary objectives and implementing model training which outcomes the core of ML systems, a model.
-
As the Domain Expert is concerned with the engineering of requirements of domain-specific undertakings, as well as validating (partial) results, this actor is irreplaceable within ML projects.
-
The assurance of ingesting real-world data in ML pipelines and optimal conditions for applying them in projects is within the responsibility of Data Stewards.
-
A Data Engineer is concerned with transforming unprocessed data sets into interpretive information for the specified systems.
-
Software Engineers achieve the requirement of deploying value-added ML-based products.
-
Operations Engineers realize the monitoring and continuous integration and delivery of the whole MLOps workflow.
-
4.1. Project Requirement Engineering
As the overall goal of a project is domain-specific, subject matters (e.g., domain experts) and data scientists must gather, engineer, and evaluate all necessary requirements for a successful implementation. One major task of the data scientist is to help the domain expert in framing the problem, such that it is feasible for being solved by ML technology [30]. As MLOps projects advocate for cross-expertise collaboration, engineering-specific obscurities originating at this point have to be clarified with the respective actors. In extreme cases, re-evaluating acquired concepts may be required, and implementing fine-grained discussions about potential uncertainties throughout the whole project becomes extremely important. By comparing scenarios with the problem on hand, possible workarounds, and the determination of human expertise required for solving them, a common understanding of how the ML system is applied in the end is generated. As the specifics of ML projects become more apparent within this process, the first draft of technologies required for successful implementation can be outlined. Next to data quality attributes, Key Performance Indicator (KPI)s of the resulting deployment, and the feasibility of the infrastructural design choices or demands on re-training of models must be identified. Additionally, much effort is required to fix the current business need for the ML solution. Business metrics differ significantly from the traditional ML metrics, and a high-performing model does not always guarantee that a higher business value would be generated.4.2. Data Management Phase
As project-specific data may be restricted by domain-dependent constants, the relational integrity of attributes, the validity of historical timelines or state-dependent transitions [34], archiving overall data quality is not an easy task. Furthermore, the life cycle of data sets is associated with different quality dimensions, as described in [23]. Data quality in ML is closely related to principles of ‘big data’. Quality issues should be discovered as early as possible in order to isolate and adapt faulty processes which impact subsequent utilization [17]. Subject-specific experts (e.g., Domain experts) are responsible for providing problem specific questions and goals, as well as KPIs for (data) models to create. The validation of potential (data as well as ML) models is in their area of responsibility, too [30]. As data scientists build operational ML models which address the formulated problem, the scientific quality must be assessed in tandem with the domain experts [30]. Depending on the organization’s structure, there are additional involved entities and roles for data operations, for example, the Data Stewardship [35], which is responsible for supervising data during various phases of a project. With a focus on Data Quality Management (DQM) and data governance, multiple data steward-related roles were defined in early approaches in [36], distinguishing between chief, business, and technical data steward, as well as a data quality board which defines the data governance framework of the organization. Especially when planning or finishing the implementation of a project, this entity is responsible for maintaining resulting data, often represented by a Data Management Plan (DMP) [37]. In [38], the stewardship was divided into three roles, where data stewards provide guidance on data governance (e.g., data integrity, provenance, requirements, and improving quality metadata), domain knowledge, and scientific integrity (e.g., data quality and usability evaluation) is provided by scientific stewards and software, and system guidance (persisting and accessing data) is provided by technical stewards. As there is a variety of tools for supporting data stewards, as well as every other involved individual, in their domain-specific work, the best practice is to gather all tooling for each experiment in a repository, accessible by all team members [39]. Concerning the quality of actual processing data, the FAIR data principles are often quoted [39] in the context of data stewards. The definition of principles for increasing reusability of data sets describes characteristics for systems with a focus on valuable research output [40]. Even when only publishing these data sets within a project, the complete data life cycle may profit from such FAIR-implementing products, such as in [37].4.2.1. Supportive Tool Requirements
With ingesting data into the system, the handling of the specific project-related data origins (e.g., sensors) must be integrated (DPR 1). In order to ensure applicable data, data preprocessing (DPR-2) and subsequent quality measures (DPR-3) are required. Due to potential massive data sets, the support of managing data (DPR-4) through cockpits is recommended.4.2.2. Science Quality
As the scientific quality of the incoming data depends on the gathered requirements of the project (see Section 4.1), data scientists and domain experts are responsible for evaluating its quality and usability [30]. From defining data accuracy and precision requirements for the intended use of information as a first critical stage, input data quality assurance, data generation quality control, and DMP are derivable during the development of input data-specific processing algorithms [23]. Next to raw data features like data types or formats, the processing of generated information (e.g., sensed or accumulated data) depends on domain-specific features like semantics, environmental influences, and statistical properties [41].4.2.3. Data Quality
Assessing the quality of a data set is a complex and multidimensional problem [23]. According to defined production workflows, data are produced by the product, e.g., a sensor. An evaluation of the actual production workflow, the produced data quality, and a comparison of the assessed quality with similar products is carried out by a domain expert in order to pinpoint data error sources, quality assurance, compliance procedures, and data processing flowcharts [23].There are multiple factors to the data quality dimensions as mentioned in [16], such as, for example, completeness of data in which problem-related missing values are considered and must be addressed. According to the authors of [17], completeness, data format and structure (e.g., consistency), accuracy (e.g., realistic values), and timeliness are the cases of the intrinsic quality dimension. To compute a data quality dimension score by applying the feasible metrics, other representational (e.g., interpretability or ease of understanding) or contextual quality dimensions like reputation or relevancy can also be measured. Additionally, ML-related quality dimensions like data dimensionality reduction, data heterogeneity, or data duplication are considered according to authors in [42].Regardless of whether the data is applied in an ML pipeline, most scenarios depend upon automated data quality checks and perform them by either incremental or batch processing of the new data.4.2.4. Stewardship Quality
In supervising rich data set metadata, its ingestion, and archiving, the overall data set quality is preserved [23] by data stewards. By ensuring metadata completeness and satisfying metadata standards and data accessibility, the data set’s maturity is evaluated, and recommendations for (re)use of this iteration can be declared. This documentation of the data sets stewardship maturity evaluation and data fixation enables a subsequent data access of well-defined data.4.2.5. Use and Service Quality
Dependent on the overall product problem, possible services must be selected while considering the provision of secure and stable interfaces for obtaining data sets, offering user support, or act as central feedback collectors. The data product may be reused for alienated purposes or being improved, based on user feedback in subsequent steps [23]. Additionally, the data stewards are required to prevent the leakage of sensitive data [11], which may require the input of domain experts in order to identify, for example, privacy risks in data usage concepts.4.3. Machine Learning Preparation Phase
This collection of functions in the ML preparation phase is mainly related to classic ML preprocessing tasks. Dependent on the previously DMP, various roles must ensure the overall data quality for the domain-specific ML solution. As data engineers are responsible for bringing the data into a ML model-consuming structure [30], support from data stewards for data ingestion is required. The overall previously defined domain-specific problem and subsequent plans for implementing the ML model also requires interaction with data scientist and domain experts.4.3.1. Supportive Tool Requirements
By preparing data for utilization by machine learning techniques, the versioning of data sets (DPR-5) is required to reference respective data origin for a specific model. As there is often no elegant way to enhance data with domain-specific information while sensing or generating them, and many use cases require support for manually labeling specific data (DPR-6).4.3.2. ML Data Acquisition
In traditional big data, the acquisition of data refers to the initial collection from sensors to the transport of data by interconnected networks to storage solution and a subsequent pre-processing (integration, enrichment, transformation, reduction, cleansing, etc.) [17]. Dependent on the product, not all collected data is relevant for the ML pipeline. Based on the previously declared DMP, a data engineer feeds the ML pipeline with an appropriate selection of the provided data. With support from data stewards, the optimal circumstances for obtaining problem-specific data are implemented.4.3.3. Data Cleaning and Labeling
Next to the elimination of existing errors in the input data, procedures for feature engineering, carried out by data scientists (in cooperation with domain experts), are necessary for other domain-specific ML operations. Data cleaning can be split into three parts [43], where error detection like duplicate data, violations of logical constraints, or incorrect value recognition is the first task. Moreover, solving every detected error is a second operation, and the data imputation supplements the missing and incomplete data as the last step.4.3.4. Data Versioning
In order to build robust and reproducible models, special attention must be payed to the versioning of every data source involved in the project. The prevention of data leakage [11], e.g., the strict separation of test, training, and validation data sets, is vital to the success of ML models When the characteristic of input data changes, the re-training of existing models, the fine-tuning of hyperparameters applied respectively may be necessary. By associating the specific circumstances, e.g., a new data version or iteration, the foundation of ’audit trails’ for input data is created. In contrast to simple ML-based approaches, where a finite set of samples trains a model, specific data versions may be extended over time with new samples or features. The advantages of versioning every piece of data involved in ML projects can be summarized as tracking the evolution of data over time. As there is often a massive amount of frequently changing input data involved in ML projects, local data repositories (e.g., edge devices) often hold the actual data set. At the same time, the remote storage solutions only persist a hash of the current version [18].4.4. ML Training Phase
This is the phase where data scientists play a significant role. These experts ensure the flexibility and scalability of ML pipelines, as well as an appropriate technology selection and efforts in model performance improvement [30]. In order to train a model until a specific goal (e.g., accuracy threshold) is reached, data scientists are responsible for choosing ML pipeline structures, algorithms and hyperparameters through model versioning and validation. They are the most expected users for the data processing phases in big data projects [17]. Every model version candidate is evaluated, and one is chosen with the domain expert for further deployment.Dependent on the required degree of automation in search for an ML pipeline, data scientists and domain experts are supported by different so-called AutoML techniques and tools. This area of ML was started to let non-technical domain experts somewhat configure the model training instead of having to implement the individual steps manually. In assembling different ML concepts and techniques, the development of feature preprocessing, model selection, and hyperparameter optimization are automated [44]. The process of selecting an algorithm, as well as its hyperparameters, is often implemented in one singular step and is referred to as CASH. In order to improve the performance in automated model training and hyperparameter optimization, techniques like k-fold cross-validation can be applied for early stopping the training when reaching a particular threshold [22].4.4.1. Supportive Tool Requirements
For implementing the actual ML training, a variety of supportive tools exist. When manual model type selection (TP-1) is required, recommendations of respective hyperparameters (TP-2) support inexperienced data scientists (as well as other individuals as non-technical domain experts) in choosing well-performing model configurations. Despite the required degree of automation while training a model, tracking the whole model run (TP-3), including quality metrics applied for validation, creates an audit trail. As there are multiple ML libraries and frameworks, many with a focus on a specific type of problem, the support for integrating them into the MLOps workflow (TP-4) is often required instead of decoupling them from the pipeline. In versioning the code for training the model (TP-5), another audit trail is created for later inspection. With packing the trained model into reproducible archives (MM-1) and their persistence in a model registry (MM-2), the foundation for ML market-place-alike structures can be set.4.4.2. Pipeline Structure Search
Dependent on the type of data (e.g., structured or unstructured input data) and the appropriate technique to solve the problem (supervised, unsupervised, or semi-supervised learning), the overall ML pipeline structure differs. As each problem probably requires its individual set of domain-specific quality demands, the regarding model performance monitoring metrics (as exemplary shown in Section 5.1.5) must be defined.4.4.3. Algorithm and Hyperparameter Selection
The selection of the most appropriate ML algorithm for a specified problem (e.g., neural networks, vector machines, and random forest) is carried out by the data scientists. On top of this, each algorithm has parameters that must be tuned for achieving good performance. These parameters are called algorithms hyperparameters, for example, the number of layers or neutrons within a neural network. Tuning these hyperparameters and selecting an optimal algorithm is one of the most resource-intensive and tedious tasks in an ML workflow. AutoML aims to simplify this and many other manual tasks in modeling by making decisions in an automated way. AutoML aims to decrease the human effort required for building efficient ML systems. It advocates for fairness and reproducibility. It supports the data scientist such that new experiments could be tried rapidly and helps the enterprise to quickly develop new ML systems through automating much of the modeling activities. A comprehensive guide on AutoML is provided in [21]. Regarding the automation of CASH which is a sub part of AutoML, different strategies (such as, for example, generating a grid of configurations and evaluating all of them or applying random search for each hyperparameter) exist [22].4.4.4. Model Version
ML models are fairly complex and have strong interdependency on data, framework, and modeling procedure. Model versioning is a way of keeping a track of these interdependencies. It is also an essential feature through which models could be rolled back if something goes wrong in production. Due to several reasons, different models could be required at different time-frames, and versioning helps in deploying the correct version at the right time. It increases accountability and works as an essential component required for governance in ML. With storing each model iteration configuration (e.g., chosen hyperparameters, data version, and quality demands) and persisting the actual versions of trained (and well performing) models, a comparable history of solutions to specific input data is created. Furthermore, the combination of model and data version makes the sharing and re-validating results in a community easy.4.4.5. Model Validation
Dependent on the input data generated at the foremost data management phase (see Section 4.2), as well as the type of implemented learning (e.g., supervised learning or unsupervised), the test and validation sets are applied for validating the learned model and ensuring the prevention of overfitting the model. As model performance is an indirect measurement of data quality applied in training [42], bad-performing models may be enhanced by fixing errors in the data management phase.4.5. Deployment Phase
The deployment phase marks the most critical phase of the MLOps journey. In this phase, software engineers integrate the validated models into the respective applications and ensure the operational stability of the whole applications system [30]. As aspects of the various decisions and configurations of the whole pipeline are derivable in this integrative phase, permanent monitoring of the model, the overall application, and consuming data must be implemented by MLOps Engineers. Another role in this infrastructural deployment phase is the DevOps engineer, which is responsible for conducting, building, and testing the working system [30].4.5.1. Supportive Tool Requirements
As the trained, validated, and registered model is ready for utilization, there are multiple application-specific and infrastructure-dependent support requirements for different deployment patterns (DP-1). As the overall system state (OMP-1) and its input data (OMP-2) will influence the overall model performance (OMP-3), constant monitoring is required. In assuring the model’s quality (OMP-4), requirements for operating the complete application are finally met.4.5.2. Solution Deployment
The sub-phase solution deployment is mainly focusing on extracted value out of the trained model. There are many potential problems and challenges in applying a trained model within existing infrastructures. Next to complications with the system-related monitoring metrics described in Section 5.1.5, various organizational or project-specific constraints, network restrictions, or missing required technologies in the ecosystem may hinder the deployment of the solution.4.5.3. Model Integration
There are three straightforward approaches of providing an application with the overvalue of an ML model, as briefly demonstrated concerning the framework ML.NET by Lee et al. in [45]. Next to deploying a model inside containers and connecting them via Remote Procedure Call (RPC) to the serving system (the application respectively), the direct integration of the model execution into the application decreases custom engineering effort during deployment. Another approach is to white-box the model, where different models are represented as Directed Acyclic graph (DAG) and registered inside a serving system which is accessible via RPC or REST calls. The exchangeability of models decreases with integrating its execution within an application’s source code. At the same time, the central deployment via REST endpoints will increase the application’s dependability on network connectivity and service availability.4.5.4. Model and Application Monitoring
The constant monitoring of the whole project stack, e.g., model and application performance, as well as infrastructural circumstances and (most importantly) the data utilized by the model, is the foundation of a robust ML-based product. In utilizing metrics suggested in Section 5.1.5, many operational errors and shortcomings can be compensated in advance. With a focus on tracking the data through the pipeline operations [11], graph databases can help in managing and maintaining linkage between data objects and the respective assertions.4.5.5. Continuous Integration and Delivery/Deployment Validation
With the goal of continuously integrating newer trained models inside the application, CI/CD Validation is the last step of the workflow in MLOps projects. To have permanent monitoring and testing of new iterations of the ML-based applications, the CI/CD validation can be applied for indicating a certain product quality and therefore is an essential component. With validating the predefined KPIs of every phase within the project, a holistic assessment of the ML-based product implementation can be derived.5. Tooling in MLOps
In recent years, many different tools have emerged which help in automating the ML pipeline. The choice of tools for MLOps is based on the context of the respective ML solution and the operations setup [24].In this section, an overview of the different requirements which these tools fulfill is discussed. Note that different tools automate different phases of the ML workflow. There is no single open-sourced tool currently known to us that can create a fully automated MLOps workflow. Specific tasks are more difficult to automate than others, such as data validation for images. Furthermore, some of the standard Full Reference-based Image Quality Assessment (FR-IQA) metrics are listed. These metrics could be applied for automating the data validation part. After discussing typical demands on such tools, 26 open-source tools are benchmarked according to these requirements. A ranking is provided indicating their fulfillment.5.1. Requirements on Tools in MLOps
In the following, various requirements for MLOps are discussed, including general demands on tooling. These set of requirements form a typical recipe based on which different tools could be selected. The requirements are based on the stages introduced in the last section. By defining these requirements as the ones that the MLOps tools must address, each can be matched to one or more of these requirements. One can identify combinations of tools that cover a range of these requirements. Furthermore, formalizing the various applicable quality metrics is out of scope for the work on hand, a brief overview of image quality, system monitoring, data monitoring, and ML system monitoring metrics is given.5.1.1. Data Preprocessing Phase (DPR)
ML models are only as good as their input data. The data collected from different sources undergo different preprocessing steps through which they are prepared to be used by an ML algorithm. There are various requirements for implementing the preprocessing steps in ML projects.DPR-1: Data Source Handling
Integrating different data sources in a project’s pipeline can be accomplished by using connectors or interfaces to load data. As every data science project depends on data sources, handling various sources (databases, files, network sockets, complete services, and many more) is vital to the pipeline.DPR-2: Data Preprocessing and Transformations
As input data are frequently formatted in incompatible ways, the preprocessing and transformation of data must be supported. Often, the domain-specific input data are of a structured nature like text or numbers. As the ML project is dependent on cleaned data, other unstructured formats like audio, images, or videos must be taken into consideration, too.DPR-3: Data Quality Measurement Support through Metrics
Automated data validation is a critical component of MLOps. In order to ensure data quality for images, one can utilize reference-based Image Quality Assessment (IQA) methods. These methods require a high-quality reference image to which other images are compared. Some of these methods are listed in Table 1. Other aspects of data quality measurements are auditioning of data (unit and integration testing for data).