Abstract
There is widespread consensus among policymakers that climate change and digitalisation constitute the most pressing global transformations shaping human life in the 21st century. Seeking to address the challenges arising at this juncture, governments, technologists and scientists alike increasingly herald artificial intelligence (AI) as a vehicle to propel climate change mitigation and adaptation. In this paper, we explore the intersection of digitalisation and climate change by examining the deployment of AI in government-led climate action. Building on participant observations conducted in the context of the “Civic Tech Lab for Green”—a government-funded public interest AI initiative—and eight expert interviews, we investigate how AI shapes the negotiation of environmental sustainability as an issue of public interest. Challenging the prescribed means–end relationship between AI and environmental protection, we argue that the unquestioned investment in AI curtails political imagination and displaces discussion of climate “problems” and possible “solutions” with “technology education”. This line of argumentation is rooted in empirical findings that illuminate three key tensions in current coproduction efforts: “AI talk vs. AI walk”, “civics washing vs. civics involvement” and “public invitation vs. public participation”. Emphasising the importance of re-exploring the innovative state in climate governance, this paper extends academic literature in science and technology studies that examines public participation in climate change adaptation by shedding light on the emergent phenomenon of public interest AI.
1 Introduction
“Like the steam engine or electricity in the past, AI is transforming our world, our society and our industry. Growth in computing power, availability of data and progress in algorithms have turned AI into one of the most strategic technologies of the 21st century. The stakes could not be higher. The way we approach AI will define the world we live in” (European Commission 2018)
Artificial intelligence (AI) will transform humanity. That is the key message from the European AI Strategy quoted above. The rise of super computing power and big data technologies, which have fuelled the latest “AI spring”, appears to have successfully captured the imagination of Western policymakers, technologists and scientists alike (Hälterlein 2024; Hine and Floridi 2024). One policy issue that is increasingly encompassed by the lure of AI constitutes environmental sustainability (Creutzig et al. 2022). Seeking to assert the interdependent nature of digitalisation and climate change, AI is heralded as providing an effective tool to propel climate change mitigation and adaptation. Clutton-Brock et al. (2021) emphasise that AI can accelerate climate action by distilling raw data into actionable information, improving predictions, optimising complex systems and accelerating scientific modelling and discovery. Key sectors in which AI is imagined to achieve climate impact include electricity systems, forestry and land use, transportation and agriculture (Kaack et al. 2022). Crucially, mobilising AI for environmental sustainability implies not simply supporting specific AI applications but also depends to a large extent on institutional capacity building. This may include implementing large-scale AI literacy and upskilling programmes for governments and civil society, funding and incentivising the creation of trusted AI for climate solution providers and auditors, as well as ensuring global access to public interest AI programmes and sharing resources across a wide variety of countries and sectors (Clutton-Brock et al. 2021; Creutzig et al. 2022).
In this paper, we explore the intersection of digitalisation and climate change by examining the investment in and deployment of AI in government-led climate action. Doing so, we adopt Suchman’s (2023) definition of AI as “a label for currently dominant computational techniques and technologies that extract statistical correlations (designated as patterns) from large datasets, based on the adjustment of relevant parameters according to either internally or externally generated feedback” (p. 2). More specifically, we zoom in on one key policy arena: public interest AI (PIAI), which denotes “AI systems that support those outcomes best serving the long-term survival and well-being of a social collective construed as a ‘public’” (Züger et al. 2022, p. 1). Building on participant observations conducted in the context of the “Civic Tech Lab for Green” (CTLG)—a German government-funded public interest AI initiative—and eight expert interviews, we investigate how the investment in AI shapes the negotiation of environmental sustainability as an issue of public interest. To this end, we engage with the following overarching research question: how does the investment in AI mediate government efforts to push civic engagement in climate change adaptation?
Challenging the prescribed means–end relationship in contemporary policy discourse between AI and environmental protection, we argue that that the unquestioned investment in AI curtails political imagination and displaces discussion of climate problems and possible solutions with “technology education”. Contemporary state-led civic tech initiatives must “decentre AI” by shifting their focus away from automation to civic data as key lever to push public participation. Existing research in science and technology studies (STS) has provided valuable insights on “public participation in climate change adaptation” (Arnstein 1969; Hügel and Davies 2020; Turnhout et al. 2020; Maas et al. 2022) and “civic technology and PIAI” (Gilman 2017; Gabrys 2019a; Züger et al. 2023). In what follows, we bring these bodies of literature into conversation using PIAI as an empirical ground for studying how citizens are engaged in co-producing knowledge for climate change adaptation. Shedding light on the politics of co-production in AI-mediated climate governance, we extend existing participation research by focussing explicitly on PIAI and contribute to civic tech literature by zooming in on a government-led PIAI initiative. More generally, this article responds to calls in STS that demand stronger diversification of and reflexivity in environmental research (Turnhout and Lahsen 2022).
This paper is structured as follows: we begin by interrogating academic literature in STS that deals with the relationship between AI, climate change adaptation and public participation. After introducing the methodology undergirding this study, we present three key empirical findings obtained from the data collection process. Building on the latter, three analytical vignettes are developed to disentangle the complex interdependencies of AI, public participation and climate change adaptation. Finally, we conclude by emphasising the importance of re-exploring the innovative state through civic data.
2 AI, environmental governance and the promise of participation
2.1 Public participation in climate change adaptation
Public participation has become a major consideration in environmental governance. It forms a critical component of contemporary efforts to combat climate change and is enshrined in key governance bodies (UNDP 2004; Lee and Romero 2023). In Article 6, the United Nations Framework on Climate Change (1992) already states that “all parties must promote and facilitate […] public participation in addressing climate change and its effects and developing adequate responses” (p. 10). Additionally, the latest IPCC (2023) report declares that “climate literacy and information provided through climate services and community approaches, including those that are informed by Indigenous Knowledge and local knowledge, can accelerate behavioural changes and planning” (p. 107). There is a long history of interdisciplinary research that investigates the role of public participation in climate change adaptation, ranging from Arnstein’s (1969) seminal “ladder of participation” in the late 1960s to the expansion of participatory processes in the 1990s (Davies 2002) to contemporary contributions on “climate action” (Corner et al. 2014; Cologna and Oreskes 2022).
Particularly relevant in the context of this paper is research that investigates the politics of co-production (Collins and Ison 2009; Turnhout et al. 2020). Whilst official policy documents and much of the literature on co-production herald public participation in environmental governance as inevitable and necessary, there is now growing academic attention on the role of power and politics in shaping participation efforts. Turnhout et al. (2020) identify three ways in which co-production processes can end up reproducing, rather than changing, existing power inequalities: (1) by a rationale of science-driven impacts that does not challenge the power of scientific knowledge over other forms of knowledge, (2) by the “tendency of co-production projects to strive for consensus and for solutions that are considered rational according to elite perspectives “ (p. 18) but cover up differences amongst less privileged groups, and finally, (3) by a lack of engagement with the wider political context in which co-production processes occur. Thus, accounts in this body of literature move beyond aspirational debates of participation as a normative ideal by grasping it as a performative practice that is inherently shaped by the socio-political context it is embedded in (Sprain 2017).
2.2 Civic technology and public interest AI
One phenomenon that links AI and climate governance constitutes civic technology (“civic tech”). Broadly defined as “technology that is explicitly leveraged to increase and deepen democratic participation “ (Gilman 2017, p. 745), civic tech rests on three pillars: transparency and accountability to hold governments accountable, effective citizen–government interaction, and digital tools that make citizens’ everyday lives easier (Dietrich 2015). Civic tech initiatives can be initiated by public institutions top-down and/or bottom-up (Sotsky and Kartt 2017). Government-led civic tech initiatives include city governments’ attempts to resist corporate control and build digital public infrastructures. The most prominent example denotes the city of Barcelona’s “New Data Deal”, which aims to reclaim data as commons and introduces procurement and data sharing standards (Fernandez-Monge et al. 2023). Examples of bottom-up civic tech initiatives include open data activists seeking to democratise datafication (Baack 2015) and civic IoT projects which collect citizen-sourced sensor data on particle matter in order to raise awareness of air pollution and influence local policy (Gabrys 2019b). One subfield of civic tech that is receiving increasing attention is PIAI. Building on Bozeman (2007) and Dewey (1927), Züger et al. (2022) define PIAI as “AI systems that support those outcomes best serving the long-term survival and well-being of a social collective construed as a ‘public’” (p. 1). Rather than individuals or corporations, the notion of public interest refers to outcomes that serve the whole community. In this context, technology is seen as a public good serving a societal benefit, for instance, “through an open democratic system of governance, with open data initiatives, open technologies, and open systems/ ecosystems designed for the collective good” (Abbas et al. 2021, p. 10).
3 Methodology
Following an interpretivist approach, this study is concerned with “how the social world is interpreted, understood, experienced, produced or constituted” (Mason 2002, p. 3), and was therefore qualitative in nature. A twofold approach was applied. Starting with ethnographic investigation of the CTLG, we conducted eight expert interviews on the basis of previously generated insights. The aim of the interviews was to triangulate previously generated ethnographic data and gain an in-depth understanding of the role of citizen participation at the intersection of AI and climate change.
3.1 Preparation and data generation of the case study “Civic Tech Lab for Green”
The CTLG—a joint initiative of the Federal Ministry for the Environment (BMUV), the Federal Ministry of Labour and Social Affairs (BMAS), and the Federal Ministry for Family Affairs, Senior Citizens, Women and Youth (BMFSFJ) in Germany—forms part of the “Civic Coding—Innovation Network AI for the Common Good”. It constitutes an initiative of the five-point programme “Artificial Intelligence for Environment and Climate”, with which the BMUV promotes the sustainable design of AI and the use of its possibilities for the benefit of the environmental protection, conservation and climate action.
Acting as an intermediary body between government, civil society and science, the CTLG explicitly focuses on citizen participation. It invites citizens to try out and experience AI technologies in order to engage with environmentalism, build competences, and produce bottom-up knowledge. Furthermore, as part of the “Civic Coding” network, it is dedicated to exploring how AI can be used for the common good. Challenging the profit motive, the CTLG emphasises the importance of safeguarding public values and advocates for ethical principles, such as participatory design, open source and sustainability.Footnote1 It employs six permanent staff covering federal territory and is supposed to facilitate discussion, offer exhibition space, provide software and hardware, support and supervise pilot projects and host a variety of sensor workshops.Footnote2 The epistemological position of this study suggested that knowledge or evidence of the chosen phenomenon could be generated by immersing oneself in “natural” or “real-life” settings (Mason 2002; Bogner 2012; Baack 2015). Keeping the main research interest in mind—civic engagement at the intersection of AI and climate change—it was helpful to not only rely on participants’ retrospective accounts but to put emphasis on situationally generated knowledge. To this end, we attended three workshops focussing on how AI can be used for environmental protection (see Figs. 1, 2, 3, 4Footnote3), specifically one on smart bee hives, one on networked trees, and one on insect protection in the city. Each workshop was attended by 5–10 people, lasted for five hours and consisted of presentations given by CTLG staff and external experts as well as group work.
3.2 Preparation and data generation of expert interviews
Furthermore, semi-structured interviews with key experts at the intersection of public interest AI and citizen participation were conducted. Keeping the qualitative nature and the ontological and epistemological assumptions underlying this research in mind, a stratified purposeful sampling strategy was adopted. This type of sampling allowed for the selection of varied and information-rich cases, thereby permitting to capture commonalities and variations amongst the chosen samples (Patton 2002). In total, eight expert interviews were conducted. Experts included one high-ranking bureaucrat heading the AI lab within the Federal Environment Agency, one executive in charge of an urban innovation lab, one individual leading a research lab on public interest AI, one person running a civic tech lab as well as
four senior academics working at the intersection of AI and sustainability (see Table 1). A semi-structured questionnaire, consisting of five parts, was developed. Afterwards, a pre-test was conducted with a researcher working in the field of civic tech, leading to further modifying identified themes of the questionnaire. The interviews, lasted between 45 and 60 min, were conducted in May and June 2023, and recorded.
3.3 Data analysis
Given the qualitative and reflexive nature of this study, the data generation and data analysis were intimately linked. The transcribing was done by use of the AI-powered audio transcription software “Trint”. In order to facilitate the organising of the generated data and to support the identification of patterns within it, the computer-assisted qualitative data analysis software NVivo 12 was used to code interview transcripts, documentary and visual material. Central to the processing of the generated data was the idea of thematic coding or categorising (Gibbs 2007). Given the exploratory nature of this research, we adopted an open coding approach. Our coding strategy consisted of two parts: (1) fieldnotes and (2) descriptive coding. Following the interview, we took fieldnotes to record immed
iate impressions and thoughts. This proved highly useful since it allowed us to reflect on emerging themes, such as tensions around defining public interest, as well as the interview flow. In a second step and following the transcription process, we conducted descriptive coding. We gathered related textual data and clustered them around “nodes” in order to identify and solidify emerging analytical themes. The latter—including conceptualisations of AI, climate knowledge-making practices and participatory design—helped challenge our initial assumptions and sharpened our analytical focus.
4 Results
4.1 Civics washing vs. civics involvement?
“Could what we are doing—similar to ethics washing and green washing—be called civics washing?” (I8, 2023)
This statement was made by an individual responsible for running the CTLG. In many ways, it captures the stark discrepancy between the promises attached to AI-enabled citizen participation on the one hand and the politics on the ground on the other. Disentangling this relationship, it makes sense to go one step back and keep the initial definition of citizen participation laid out above in mind. According to that, there are two main components that shape i
ts implementation: knowledge creation and empowerment (I3, 2023). What kinds of knowledge are transferred at urban labs? Who exactly is empowered? Describing the purpose of urban labs, interviewees kept emphasising the “learning by making” culture. One of the architects behind the CTLG stresses that “we want to enable the experts, we want to enable the people who come to us to ultimately also maintain, promote and further develop their solutions and maybe also see where there are coupling effects” (I1, 2023).
One of the key means of achieving this mission are sensor workshops. Taking place on the first Saturday of every month with varying thematic foci, they are directed at organised civil society, i.e. people with prior knowledge and experience in environmental movements. This specification is important since it hints at tensions which emerged in the very conception phase of the CTLG. Whilst the BMUV saw the CTLG’s mission as serving primarily as a platform for technological innovation—equipping organised civil society with data science skills—the staff running the CTLG pursued a different goal:
“That is, this networking, being the platform for social innovation, that is the subtitle of o
ur mission, which we as a team of experts have taken very, very seriously. Politically, this is not wanted, because the term social innovation is associated with another ministry and with another party. […] Why should I, as an environmental activist, propose to you now a technology that may be obsolete in ten years or that has side effects in production?” (I8, 2023)
The quote above demonstrates that the very design of citizen engagement efforts is intimately linked to political agendas. In other words, different actors “own” different terminologies. The “social” in innovation is occupied by the BMAS whilst the “technological” dimension is advanced by the BMUV. The employees of the CTLG therefore find themselves in a context of conflicting interests. For a start, CTLG staff received no training with regard to running effective participation formats (I8, 2023). Put differently, those individuals supposed to foster civic engagement and empower civil society had to rely solely on existing community engagement skills. One interviewee emphasises that prior training in running effective participation formats is absolutely crucial for ensuring the sustainability of civic engagement projects (I3, 2023).
A second observation refers to the people who attended the workshops. Given the setting—first Saturday of every month, indoors, running from 10 am to 3 pm—attendance remained sparse. Mirroring insights from similar, government-led participation efforts (I3, 2023), the workshops attracted mainly white, male and tech-savvy individuals. Whilst not particularly surprising, one can’t help but think of the enormous potential of CTLG’s site. Located in the heart of Berlin-Neukölln—a highly mixed neighbourhood with a range of socioeconomic inequalities—a different, more inclusive approach would promise to reach much more diverse audiences. Reflecting on the conflicting objectives guiding the mission of the CTLG, one staff member critically remarks:
“I also ask myself the question quite openly and quite honestly, and I would also put it on the record. So feel free to quote me on that. Are we really living up to our claim? And if civil society comes along and we say “no” every time, really every time. We have
n’t even said “yes” anywhere, but “no” every time, then we lose our credibility as people who work there, who have a genuine interest, who work there for that reason” (I8, 2023)
Interestingly, those who attended the workshops did not identify themselves as “citizen scientists” but rather as “civic tech activists” curious about exploring technological solutions in the context of climate change (Pfitzner-Eden and Samoilova 2023). With backgrounds in design, public administration, data science and experiences in the “quantified self” movement, what prevailed was a sense of technological solutionism (Morozov 2013). This can be attribut
ed to the CTLG’s ambiguous positioning at the boundary of deterministic and critical understandings of innovation. On the one hand, the design of the lab feeds into the belief that technologies such as AI can “solve” complex issues such as the climate crisis. On the other, t
hose running the CTLG advocate a view on technology that directly challenges tech-solutionist claims.
4.2 Public invitation vs. public participation
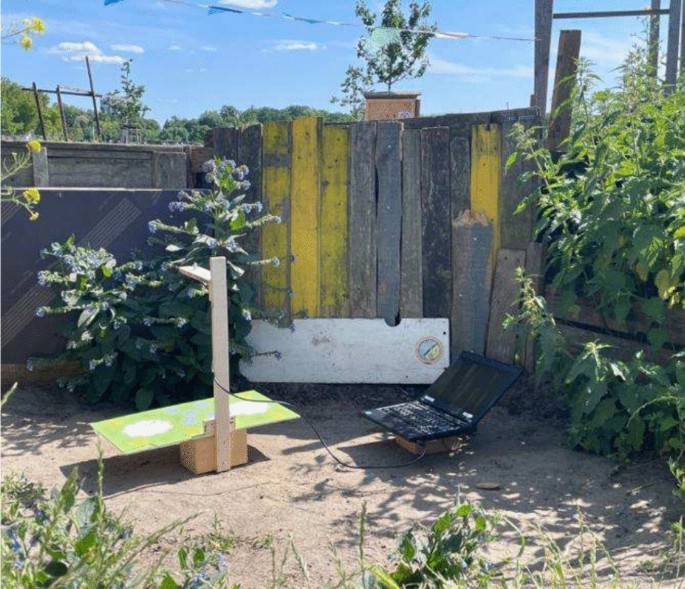
The empirical insights presented above seem emblematic of not just the CTLG but reflect broader concerns regarding the role of citizen participation in civic tech initiatives. On the one hand, there was broad consensus amongst interviewees that public participation is valuable, both intrinsically, as a means of democratic expression and procedural justice, and instrumentally, as a means of increasing political accountability and securing trust in governance processes. This senior researcher at an urban science lab points out:
“And that’s why I consider any form of participation of the people who live and work in the city, in this multi-stakeholder approach, i.e. the involvement of different interest groups, different people in very different contexts, but above all the joint development with them, absolutely necessary when it comes to developing cities worth living in. To do this you need people, and people know that, and also the possibility that these people can create something. That’s why participation is ultimately the basis for our work” (I4, 2023)
At the same time, respondents expressed considerable doubts as to the design and execution of current participation formats. This interviewee remarks:
“So I think it’s true that I’m also sceptical about many current forms of participation. But not because I think participation is somehow stupid per se, but because I have the impression that it is not done well and that it quickly becomes a fig leaf story or token participation. In part, it’s just the typical diffusion of responsibility into a process” (I5, 2023)
This insight is important because it illuminates the difficulties involved in establishing effective citizen participation. More generally, it challenges the assumption that civic engagement inevitably advances more inclusive technology development. A further insight that was persistently highlighted refers to the ways in which citizens are approached. Reflecting on what effective civic engagement can look like, this public interest AI expert emphasises that “[…] if you want this exchange with citizens, then you usually have to go to them and actively consider it beforehand […]” (I7, 2023). In other words, it is not enough to simply “invite” citizens to participate. Instead, successful civic engagement requires community building, i.e. meeting and engaging citizens in their “natural habitats” (I3, 2023).
Finally, respondents highlighted inherent tensions in existing knowledge-making practices. Confronted with the observation that digitalisation involves not simply the introduction and proliferation of digital technologies but also changes in underlying epistemic infrastructures, one third sector expert emphasised the importance of first making existing knowledge-making processes more transparent, which will then eventually ensure more effective social innovation. Reflecting on current standards and processes in information technology infrastructure of a public administration, one interviewee urges:
“It’s a huge mess. It is highly fragmented and decentralized and there are many people in the administration with partial secret knowledge, but this is not written down anywhere. And it really takes years of painstaking work to create these networks. And that’s a really big interest of ours […] to create visibility, for example to write down which steps actually have to be followed and in what order to actually launch an innovative product in Berlin.” (I5, 2023)
4.3 AI talk vs. AI walk
Another finding refers to the role of AI in mediating the co-production of climate change knowledge. Whilst the CTLG is explicitly built around AI, one couldn’t help but notice the strong discrepancy between “AI talk” and “AI walk”. Put differently, AI featured first and foremost verbally rather than materially. The point being that most of the group activities were concerned with identifying relevant sources of data, setting up data collection processes via sensor technologies and establishing an IoT ecosystem instead of deploying AI in data analysis. The absence of AI became particularly clear during the second sensor workshop when a discussion erupted around the societal impact of AI. What is the difference between AI and machine learning? How does a neural network function? What are the implications and limits of generative AI models such as ChatGPT? What does intelligence mean in the first place? (see Figs. 5 and 6) This insight is crucial since it illuminates the importance of clarifying what AI is in the first place before assuming that it can be deployed as a tool for environmentalism.