Recent years have seen impressive advances in artificial intelligence (AI) and this has stoked renewed concern about the impact of technological progress on the labor market, including on worker displacement. This paper looks at the possible links between AI and employment in a cross-country context. It adapts the AI occupational impact measure developed by Felten, Raj and Seamans—an indicator measuring the degree to which occupations rely on abilities in which AI has made the most progress—and extends it to 23 OECD countries. Overall, there appears to be no clear relationship between AI exposure and employment growth. However, in occupations where computer use is high, greater exposure to AI is linked to higher employment growth. The paper also finds suggestive evidence of a negative relationship between AI exposure and growth in average hours worked among occupations where computer use is low. One possible explanation is that partial automation by AI increases productivity directly as well as by shifting the task composition of occupations toward higher value-added tasks. This increase in labor productivity and output counteracts the direct displacement effect of automation through AI for workers with good digital skills, who may find it easier to use AI effectively and shift to non-automatable, higher-value added tasks within their occupations. The opposite could be true for workers with poor digital skills, who may not be able to interact efficiently with AI and thus reap all potential benefits of the technology1.
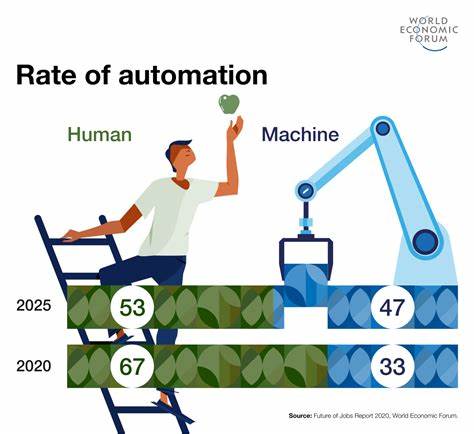
Introduction
Recent years have seen impressive advances in Artificial Intelligence (AI), particularly in the areas of image and speech recognition, natural language processing, translation, reading comprehension, computer programming, and predictive analytics.
This rapid progress has been accompanied by concern about the possible effects of AI deployment on the labor market, including on worker displacement. There are reasons to believe that its impact on employment may be different from previous waves of technological progress. Autor et al. (2003) postulated that jobs consist of routine (and thus in principle programmable) and non-routine tasks. Previous waves of technological progress were primarily associated with the automation of routine tasks. Computers, for example, are capable of performing routine cognitive tasks including record-keeping, calculation, and searching for information. Similarly, industrial robots are programmable manipulators of physical objects and therefore associated with the automation of routine manual tasks such as welding, painting or packaging (Raj and Seamans, 2019)2. These technologies therefore mainly substitute for workers in low- and middle-skill occupations.
Tasks typically associated with high-skilled occupations, such as non-routine manual tasks (requiring dexterity) and non-routine cognitive tasks (requiring abstract reasoning, creativity, and social intelligence) were previously thought to be outside the scope of automation (Autor et al., 2003; Acemoglu and Restrepo, 2020).
However, recent advances in AI mean that non-routine cognitive tasks can also increasingly be automated (Lane and Saint-Martin, 2021). In most of its current applications, AI refers to computer software that relies on highly sophisticated algorithmic techniques to find patterns in data and make predictions about the future. Analysis of patent texts suggests AI is capable of formulating medical prognosis and suggesting treatment, detecting cancer and identifying fraud (Webb, 2020). Thus, in contrast to previous waves of automation, AI might disproportionally affect high-skilled workers.
Even if AI automates non-routine, cognitive tasks, this does not necessarily mean that AI will displace workers. In general, technological progress improves labor efficiency by (partially) taking over/speeding up tasks performed by workers. This leads to an increase in output per effective labor input and a reduction in production costs. The employment effects of this process are ex-ante ambiguous: employment may fall as tasks are automated (substitution effect). On the other hand, lower production costs may increase output if there is sufficient demand for the good/service (productivity effect)3.
To harness this productivity effect, workers need to both learn to work effectively with the new technology and to adapt to a changing task composition that puts more emphasis on tasks that AI cannot yet perform. Such adaptation is costly and the cost will depend on worker characteristics.
The areas where AI is currently making the most progress are associated with non-routine, cognitive tasks often performed by medium- to high-skilled, white collar workers. However, these workers also rely more than other workers on abilities AI does not currently possess, such as inductive reasoning or social intelligence. Moreover, highly educated workers often find it easier to adapt to new technologies because they are more likely to already work with digital technologies and participate more in training, which puts them in a better position than lower-skilled workers to reap the potential benefits of AI. That being said, more educated workers also tend to have more task-specific human capital4, which might make adaption more costly for them (Fossen and Sorgner, 2019).
As AI is a relatively new technology, there is little empirical evidence on its effect on the labor market to date. The literature that does exist is mostly limited to the US and finds little evidence for AI-driven worker displacement (Lane and Saint-Martin, 2021). Felten et al. (2019) look at the effect of exposure to AI5 on employment and wages in the US at the occupational level. They do not find any link between AI exposure and (aggregate) employment, but they do find a positive effect of AI exposure on wage growth, suggesting that the productivity effect of AI may outweigh the substitution effect. This effect on wage growth is concentrated in occupations that require software skills and in high-wage occupations.
Again for the US, Fossen and Sorgner (2019) look at the effect of exposure to AI6 on job stability and wage growth at the individual level. They find that exposure to AI leads to higher employment stability and higher wages, and that this effect is stronger for higher educated and more experienced workers, again indicating that the productivity effect dominates and that it is stronger for high-skilled workers.
Finally, Acemoglu et al. (2020) look at hiring in US firms with task structures compatible with AI capabilities7. They find that firms’ exposure to AI is linked to changes in the structure of skills that firms demand. They find no evidence of employment effects at the occupational level, but they do find that firms that are exposed to AI restrict their hiring in non-AI positions compared to other firms. They conclude that the employment effect of AI might still be too small to be detected in aggregate data (given also how recent a phenomenon AI is), but that it might emerge in the future as AI adoption spreads.
This paper adds to the literature by looking at the links between AI and employment growth in a cross-country context. It adapts the AI occupational impact measure proposed by Felten et al. (2018, 2019)—an indicator measuring the degree to which occupations rely on abilities in which AI has made the most progress in recent years—and extends it to 23 OECD countries by linking it to the Survey of Adult Skills, PIAAC. This indicator, which allows for variations in AI exposure across occupations, as well as within occupations and across countries, is matched to Labor Force Surveys to analyse the relationship with employment growth.
The paper finds that, over the period 2012–2019, there is no clear relationship between AI exposure and employment growth across all occupations. Moreover, in occupations where computer use is high, AI appears to be positively associated with employment growth. There is also some evidence of a negative relationship between AI exposure and growth in average hours worked among occupations where computer use is low. While further research is needed to identify the exact mechanisms driving these results, one possible explanation is that partial automation by AI increases productivity directly as well as by shifting the task composition of occupations toward higher value-added tasks. This increase in labor productivity and output counteracts the direct displacement effect of automation through AI for workers with good digital skills, who may find it easier to use AI effectively and shift to non-automatable, higher-value tasks within their occupations. The opposite could be true for workers with poor digital skills, who may be unable to interact efficiently with AI and thus reap all potential benefits of the technology.
The paper starts out by presenting indicators of AI deployment that have been proposed in the literature and discussing their relative merits (Section Indicators of Occupational Exposure to AI). It then goes on to present the indicator developed in this paper and builds some intuition on the channels through which occupations are potentially affected by AI (Section Data). Section Results presents the main results.
Indicators of Occupational Exposure to AI
To analyse the links between AI and employment, it is necessary to determine where in the economy AI is currently deployed. In the absence of comprehensive data on the adoption of AI by firms, several proxies for (potential) AI deployment have been proposed in the literature. They can be grouped into two broad categories. The first group of indicators uses information on labor demand to infer AI activity across occupations, sectors and locations. In practice, these indicators use online job postings that provide information on skills requirements and they therefore will only capture AI deployment if it requires workers to have AI skills. The second group of indicators uses information on AI capabilities—that is, information on what AI can currently do—and links it to occupations. These indicators measure potential exposure to AI and not actual AI adoption. This section presents some of these indicators and discusses their advantages and drawbacks.
Indicators Based on AI-Related Job Posting Frequencies
The first set of indicators use data on AI-related skill requirements in job postings as a proxy for AI deployment in firms. The main data source for these indicators is Burning Glass Technologies (BGT), which collects detailed information—including job title, sector, required skills etc. —on online job postings (see Box 1 for details). Because of the rich and up-to-date information BGT data offers, these indicators allow for a timely tracking of the demand for AI skills across the labor market.
Box 1. Burning Glass Technologies (BGT) online job postings data
Burning Glass Technologies (BGT) collects data on online job postings by web-scraping 40 000 online job boards and company websites. It claims to cover the near-universe of online job postings. Data are currently available for Australia, Canada, New Zealand, Singapore, the United Kingdom, and the United States for the time period 2012–2020 (2014–2020 for Germany and 2018–2020 for other European Union countries). BGT extracts information such as location, sector, occupation, required skills, education, and experience levels from the text of job postings (deleting duplicates) and organizes it into up to 70 variables that can be linked to labor force surveys, providing detailed, and timely information on labor demand.
Despite its strengths, BGT data has a number of limitations:
• It misses vacancies that are not posted online. Carnevale et al. (2014) compare vacancies from survey data according to the Job Openings and Labor Turnover Survey (JOLTS) from the US Bureau of Labor Statistics, a representative survey of 16,000 US businesses, with BGT data for 2013. They find that roughly 70% of vacancies were posted online, with vacancies requiring a college degree significantly more likely to be posted online compared to jobs with lower education requirements.
• There is not necessarily a direct, one-to-one correspondence between an online job ad and an actual vacancy: firms might post one job ad for several vacancies, or post job ads without firm plans to hire, e.g., because they want to learn about available talent for future hiring needs.
• BGT data might over-represent growing firms that cannot draw on internal labor markets to the same extent as the average firm.
• Higher turnover in some occupations and industries can produce a skewed image of actual labor demand since vacancies reflect a mixture of replacement demand as well as expansion.
In addition, since BGT data draws on published job advertisements, it is a proxy of current vacancies, and not of hiring or actual employment. As a proxy for vacancies, BGT data performs reasonably well, although some occupations and sectors are over-represented. Hershbein and Kahn (2018) show for the US that, compared to vacancy data from the U.S. Bureau of Labor Statistics’ Job Openings and Labor Turnover Survey (JOLTS), BGT over-represents health care and social assistance, finance and insurance, and education, while under-representing accommodation, food services and construction (where informal hiring is more prevalent) as well as public administration/government. These differences are stable across time, however, such that changes in labor demand in BGT track well with JOLTS data. Regarding hiring, they also compare BGT data with new jobs according to the Current Population Survey (CPS). BGT data strongly over-represents computer and mathematical occupations (by a factor of over four, which is a concern when looking at growth in demand for AI skills as compared to other skills), as well as occupations in management, healthcare, and business and financial operations. It under-represents all remaining occupations, including transportation, food preparation and serving, production, or construction.
Cammeraat and Squicciarini (2020) argue that, because of differences in turnover across occupations, countries and time, as well as differences in the collection of national vacancy statistics, the representativeness of BGT data as an indicator for labor and skills demand should be measured against employment growth. They compare growth rates in employment with growth rates in BGT job postings on the occupational level in the six countries for which a BGT timeline exists. They find that, across countries, the deviation between BGT and employment growth rates by occupation is lower than 10 percentage points for 65% of the employed population. They observe the biggest deviations for agricultural, forestry and fishery workers, as well as community and personal service workers, again occupations where informal hiring may be more prevalent.
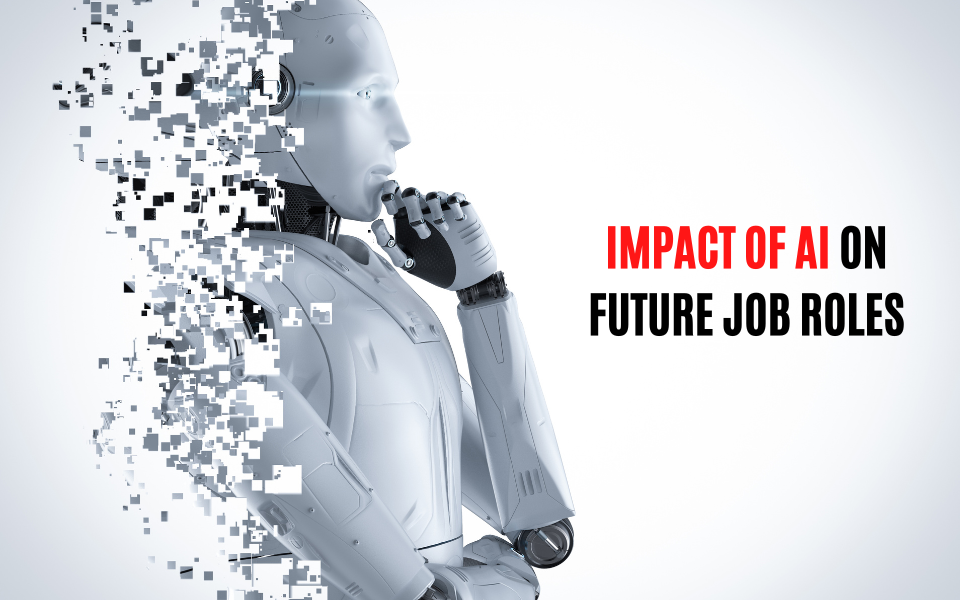
Squicciarini and Nachtigall (2021) identify AI-related job postings by using keywords extracted from scientific publications, augmented by text mining techniques and expert validation [see Baruffaldi et al. (2020) for details]. These keywords belong to four broad groups: (i) generic AI keywords, e.g., “artificial intelligence,” “machine learning;” (ii) AI approaches or methods: e.g., “decision trees,” “deep learning;” (iii) AI applications: e.g., “computer vision,” “image recognition;” (iv) AI software and libraries: e.g., Python or TensorFlow. Since some of these keywords may be used in job postings for non AI-related jobs (e.g., “Python” or “Bayesian”), the authors only tag a job as AI-related if the posting contains at least two AI keywords from at least two distinct concepts. This indicator is available on an annual basis for Canada, Singapore, the United Kingdom and the United States, for 2012–20188.
Acemoglu et al. (2020) take a simpler approach by defining vacancies as AI-related if they contain any keyword belonging to a simple list of skills related to AI9. As this indicator will tag any job posting that contains one of the keywords, it is less precise than the indicator proposed by Squicciarini and Nachtigall (2021), but also easier to reproduce.
Dawson et al. (2021) develop the skills-space or skills-similarity indicator. This approach defines two skills as similar if they often occur together in BGT job postings and are both simultaneously important for the job posting. A skill is assumed to be less “important” for a particular job posting if it is common across job postings. For example, “communication” and “team work” occur in about a quarter of all job adds, and would therefore be less important than “machine learning” in a job posting requiring both “communication” and “team work10.” The idea behind this approach is that, if two skills are often simultaneously required for jobs, (i) they are complementary and (ii) mastery of one skill means it is easier to acquire the other. In that way, similar skills may act as “bridges” for workers wanting to change occupations. It also means that workers who possess skills that are similar to AI skills may find it easier to work with AI, even if they are not capable of developing the technology themselves. For example, the skill “copy writing” is similar to “journalism,” meaning that a copy writer might transition to journalism at a lower cost than, say, a social worker, and that a copy writer might find it comparatively easier to use databases and other digital tools created for journalists.
Skill similarity allows the identification and tracking of emerging skills: using a short list of “seed skills11,” the indicator can track similar skills as they appear in job ads over time, keeping the indicator up to date. For example, TensorFlow is a deep learning framework introduced in 2016. Many job postings now list it as a requirement without additionally specifying “deep learning” (Dawson et al., 2021).
The skill similarity approach is preferable to the simple job posting frequency indicators mentioned above (Acemoglu et al., 2020; Squicciarini and Nachtigall, 2021) as it does not only pick up specific AI job postings, but also job postings with skills that are similar (but not identical) to AI skills, and may thus enable workers to work with AI technologies. Another advantage of this indicator is its dynamic nature: as technologies develop and skill requirements evolve, skill similarity can identify new skills that appear in job postings together with familiar skills, and keep the relative skill indicators up-to-date. This indicator is available at the annual level from 2012 to 2019 for Australia and New Zealand12.
Task-Based Indicators
Task-based indicators for AI adoption are based on measures of AI capabilities linked to tasks workers perform, often at the occupational level. They identify occupations as exposed to AI if they perform tasks that AI is increasingly capable of performing.
The AI occupational exposure measure developed by Felten et al. (2018, 2019) is based on progress scores in nine AI applications13 (such as reading comprehension or image recognition) from the AI progress measurement dataset provided by the Electronic Frontier Foundation (EFF). The EFF monitors progress in AI applications using a mixture of academic literature, blog posts and websites focused on AI. Each application may have several progress scores. One example of a progress score would be a recognition error rate for image recognition. The authors rescale these scores to arrive at a composite score that measures progress in each application between 2010 and 2015.
Felten et al. (2018, 2019) then link these AI applications to abilities in the US Department of Labor’s O*NET database. Abilities are defined as “enduring attributes of the individual that influence performance,” e.g., “peripheral vision” or “oral comprehension.” They enable workers to perform tasks in their jobs (such as driving a car or answering a call), but are distinct from skills in that they cannot typically be acquired or learned. Thus, linking O*NET abilities to AI applications means linking human to AI abilities.
The link between O*NET abilities and AI applications (a correlation matrix) is made via an Amazon Mechanical Turk survey of 200 gig workers per AI application, who are asked whether a given AI application—e.g., image recognition—can be used for a certain ability—e.g., peripheral vision14. The correlation matrix between applications and abilities is then calculated as the share of respondents who thought that a given AI application could be used for a given ability. These abilities are subsequently linked to occupations using the O*NET database. This indicator is available for the US for 2010–201515.
Similarly, the Suitability for Machine Learning indicator developed by Brynjolfsson and Mitchell (2017); Brynjolfsson et al. (2018) assigns a suitability for machine learning score to each of the 2,069 narrowly defined work activities from the O*NET database that are shared across occupations (e.g., “assisting and caring for others,” “coaching others,” “coordinating the work of others”). For these scores, they use a Machine Learning suitability rubric consisting of 23 distinct statements describing a work activity. For example, for the statement “Task is describable by rules,” the highest score would be “Task can be fully described by a detailed set of rules (e.g., following a recipe),” whereas the lowest score would be “The task has no clear, well-known set of rules on what is and is not effective (e.g., writing a book).” They use the human intelligence task crowdsourcing platform CrowdFlower to score each direct work activity by seven to ten respondents. The direct work activities are then aggregated to tasks (e.g., “assisting and caring for others,” “coaching others,” “coordinating the work of others” aggregate to “interacting with others”), and the tasks to occupations. This indicator is available for the US for the year 2016/2017.
Tolan et al. (2021) introduce a layer of cognitive abilities to connect AI applications (that they call benchmarks) to tasks. The authors define 14 cognitive abilities (e.g., visual processing, planning and sequential decision-making and acting, communication, etc.) from the psychometrics, comparative psychology, cognitive science, and AI literature16. They link these abilities to 328 different AI benchmarks (or applications) stemming from the authors’ own previous analysis and annotation of AI papers as well as from open resources such as Papers with Code17. These sources in turn draw on data from multiple verified sources, including academic literature, review articles etc. on machine learning and AI. They use the research intensity in a specific benchmark (number of publications, news stories, blog entries etc.) obtained from AI topics18. Tasks are measured at the worker level using the European Working Conditions Survey (EWCS), PIAAC and the O*NET database. Task intensity is derived as a measure of how much time an individual worker spends on a task and how often the task is performed.
The mapping between cognitive abilities and AI benchmarks, as well as between cognitive abilities and tasks, relies on a correspondence matrix that assigns a value of 1 if the ability is absolutely required to solve a benchmark or complete a task, and 0 if it is not necessary at all. This correspondence matrix was populated by a group of multidisciplinary researchers for the mapping between tasks and cognitive abilities, and by a group of AI-specialized researchers for the mapping between AI benchmarks and cognitive abilities. This indicator is available from 2008 to 2018, at the ISCO-3 level, and constructed to be country-invariant (as it combines data covering different countries).
Webb (2020) constructs his exposure of occupations to any technology indicator by directly comparing the text of patents from Google patents public data to the texts of job descriptions from the O*NET database to quantify the overlap between patent descriptions and job task descriptions. By limiting the patents to AI patents (using a list of key-words), this indicator can be narrowed to only apply to AI. Each particular task is then assigned a score according to the prevalence of such patents that mention this task; tasks are then aggregated to occupations.
What Do These Indicators Measure?
To gauge the link between AI and employment, the chosen indicator for this study should proxy actual AI deployment in the economy as closely as possible. Furthermore, it should proxy AI deployment at the occupation level because switching occupations is more costly for workers than switching firms or sectors, making the occupation the relevant level for the automation risk of individual workers.
Task-based approaches measure potential automatability of tasks (and occupations), so they are measures of AI exposure, not deployment. Because task-based measures look at potential automatability, they cannot capture uneven adoption of AI across occupations, sectors or countries. Thus, in a cross-country analysis, the only source of variation in a task-based indicator are differences in the occupational task composition across countries, as well as cross-country differences in the occupational distribution.
Indicators based on job posting data measure demand for AI skills (albeit with some noise, see Box 1), as opposed to AI use. Thus, they rely on the assumption that AI use in a firm, sector or occupation will lead to employer demand for AI skills in that particular firm, sector, or occupation. This is not necessarily the case, however:
• Some firms will decide to train workers in AI rather than recruit workers with AI skills; their propensity to do so may vary across occupations.
• Many AI applications will not require AI skills to work with them.
• Even where AI skills are needed, many firms, especially smaller ones, are likely to outsource AI development and support with its adoption to specialized AI development firms. In this case, vacancies associated with AI adoption would emerge in a different firm or sector to where the technology was actually being deployed.
• The assumption that AI deployment requires hiring of staff with AI skills is even more problematic when the indicator is applied at the occupation level. Firms that adopt AI may seek workers with AI skills in completely different occupations than the workers whose tasks are being automated by AI. For instance, an insurance company wanting to substitute or enhance some of the tasks of insurance clerks with AI would not necessarily hire insurance clerks with AI skills, but AI professionals to develop or deploy the technology. Insurance clerks may only have to interact with this technology, which might not require AI development skills (but may well-require other specialized skills). Thus, even with broad-based deployment of AI in the financial industry, this indicator may not show an increasing number of job postings for insurance clerks with AI skills. This effect could also be heterogeneous across countries and time. For example, Qian et al. (2020) show that law firms in the UK tend to hire AI professionals without legal knowledge, while law firms in Singapore and the US do advertise jobs with hybrid legal-AI skillsets.
Thus, indicators based on labor demand data are a good proxy for AI deployment at the firm and sector level as long as there is no significant outsourcing of AI development and maintenance, and the production process is such that using the technology requires specialized AI skills. If these assumptions do not hold, these indicators will be incomplete. Whether or not this is the case is an empirical question that requires further research. To date the only empirical reference on this question is Acemoglu et al. (2020) who show for the US that the share of job postings that require AI skills increases faster in firms that are heavily exposed to AI (according to task-based indicators). For example, a one standard deviation increase in the measure of AI exposure according to Felten et al. (2018, 2019) leads to a 15% increase in the number of published AI vacancies.
To shed further light on the relationship between the two types of indicators, Figure 1 plots the 2012–2019 percentage point change in the share of BGT job postings that require AI skills19 across 36 sectors against a sector-level task-based AI exposure score, similar to the occupational AI exposure score developed in this paper (see Section Construction of the AI Occupational Exposure Measure)20. This analysis only covers the United Kingdom and the United States21 because of data availability. For both countries, a positive relationship is apparent, suggesting that, overall, (i) the two measures are consistent and (ii) AI deployment does require some AI talent at the sector level. Specifically, a one standard deviation increase in AI exposure (approximately the difference in exposure between finance and public administration) is associated with a 0.33 higher percentage point change in the share of job postings that require AI skills in the United-Kingdom; a similar relationship emerges in the United-States22.